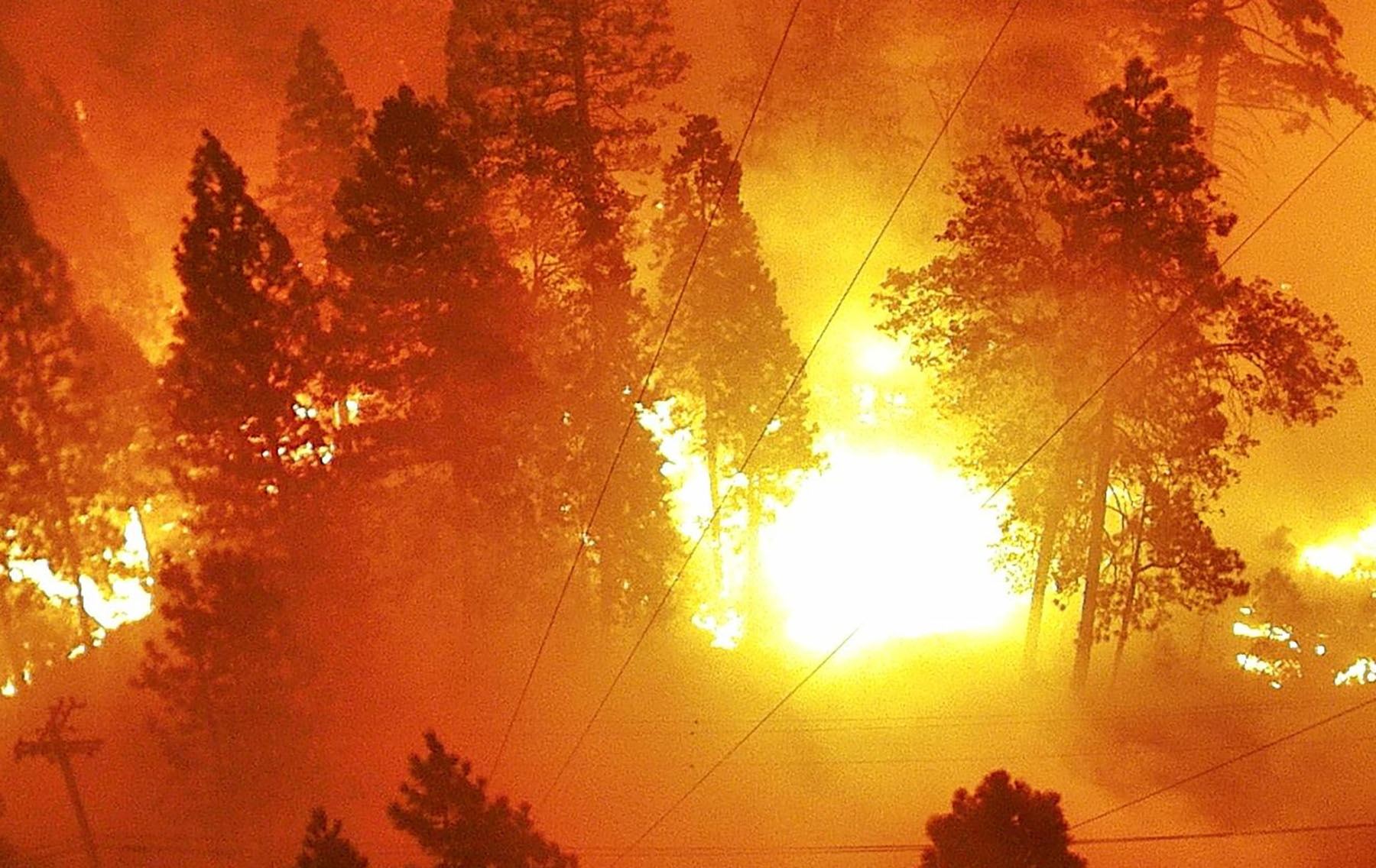
Climate scientists and fire agencies looking to get ahead of evolving fire behavior have begun to leverage artificial intelligence in wildfire detection.
In addition to speeding up response times, these systems feed back into fire-propagation models that may help engineers as they plan along the growing wildland-urban interface. These advances could prove critical to keeping people and infrastructure safer from wildfires.
The urgency of detection
Detection time remains one of the major levers to improve wildfire outcomes.
“Unless we know about it, there’s nothing we can do,” said Falko Kuester, Ph.D., an engineering professor at the University of California San Diego. He cited the Apple Fire, which burned 33,000 acres in Southern California in 2020, to illustrate how time is of the essence with wildland fires. A retrospective of camera feeds at the time showed the fire progressing from onset to full-on “apocalyptic fire” inside 40 minutes, he continues.
Further reading:
- Model estimates 30-year wildfire risks for US properties
- How civil engineers can help with wildfire prevention and preparedness
- How engineers combat conditions within the ‘red zone’ of global warming
With shorter detection times, fire agencies can shift from a defensive stance of protecting actively threatened lives and property to an offensive one, where they can proactively manage a wildfire’s path before it becomes uncontrollable, Kuester says.
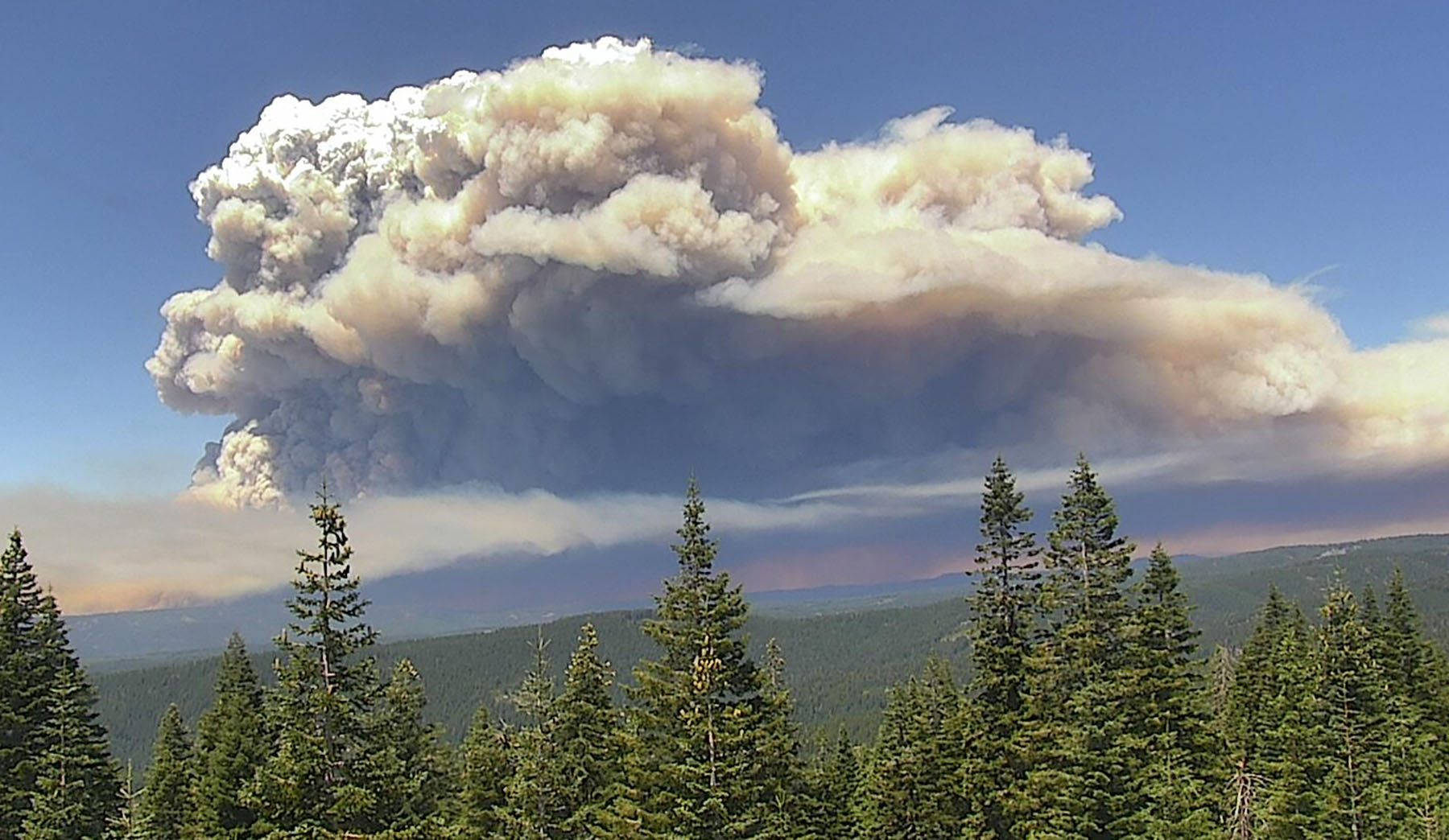
Kuester is the co-principal investigator for ALERTCalifornia, a public safety program that includes 1,100 tower-mounted pan-tilt-zoom cameras across California. An evolution of a sensor system originally intended for geologic monitoring, the ALERTCalifornia network streams an enormous amount of data – roughly three gigapixels of images per second – that would quickly overwhelm a team of human-only operators. AI algorithms are trained to identify the smoke plumes that deserve human attention.
“AI does the first heavy lift – taking a thousand feeds and condensing it down to a couple that are tracking information of interest,” Kuester said. “Then the subject matter expert goes in and labels it, and from that, they can then decide how to marshal resources.”
The system pushes anomalies to fire agencies like the California Department of Forestry and Fire Protection, which then gain control of where the cameras are looking.
Traditionally, detection has relied on human observations and public reports – a lucky catch from a commercial airliner, for example. Such reports not only need fires to first grow beyond a certain threshold but also provide little information to decision-makers, compounding delays.
“These delays allow fires to grow uncontrollably, increasing the risk to communities and the environment,” says Sonia Kastner, co-founder and CEO of San Francisco-based Pano AI.
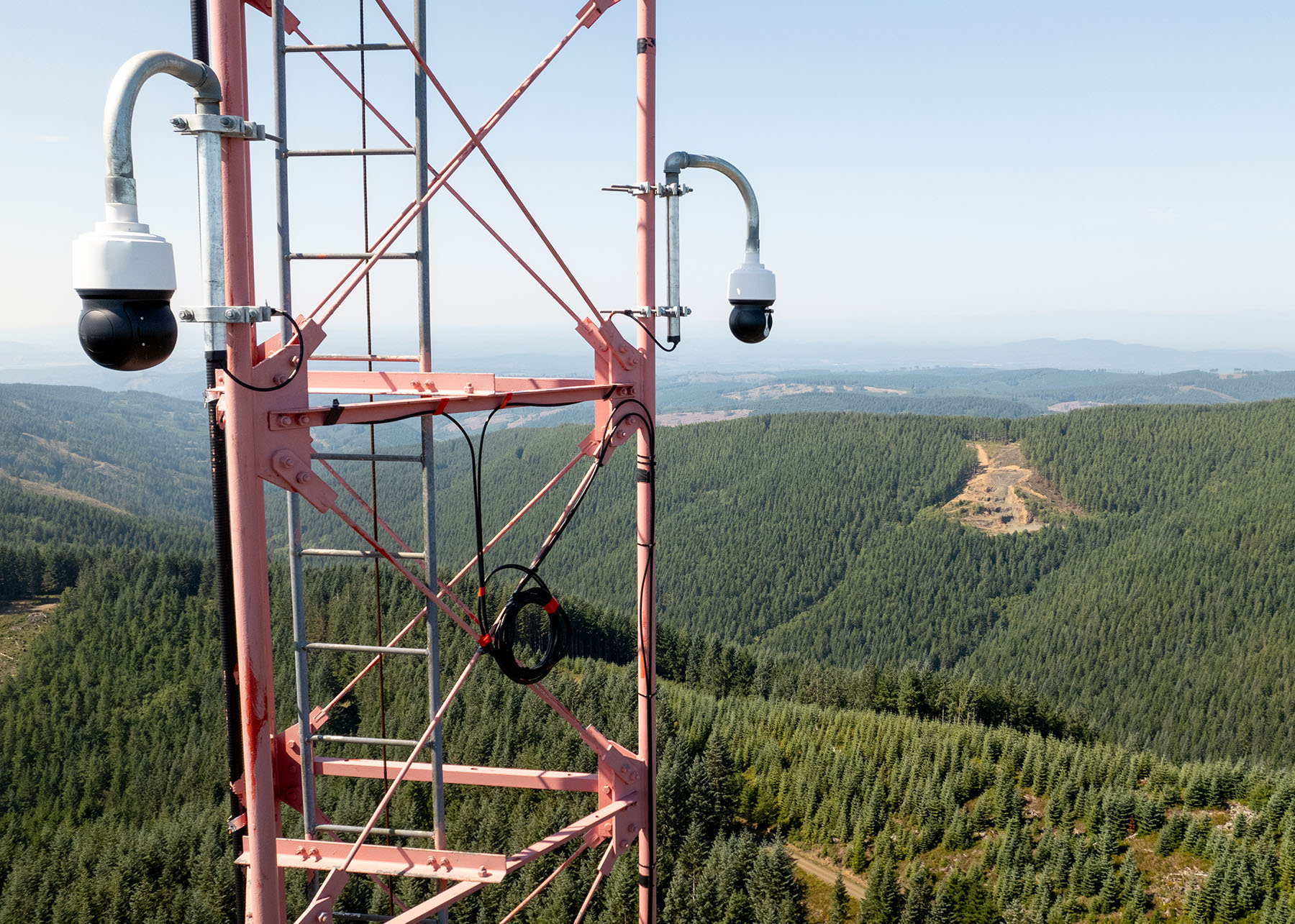
Pano, founded in 2019, is one of several startups leaning on AI for wildfire detection. Its system, Pano Rapid Detect, aggregates ultra-high-definition cameras, geo satellite data, field sensors, legacy cameras, emergency alerts, and other data feeds to detect fires faster. It has been deployed to assist fire agencies battling blazes in 10 states across the western United States, five states in Australia, and one Canadian province. Pano has also partnered with utility companies – such as Portland (Oregon) General Electric, Xcel Energy Colorado, Austin (Texas) Energy, and Holy Cross Energy – to protect infrastructure.
The system, for example, assisted with the 2023 Jackson Road Fire in Washington state by alerting agencies within minutes of onset and reducing the time to put resources on-site by 20-30 minutes, Kastner says.
“(The dispatch manager) said early notice to (the Washington State Department of Natural Resources) 100% impacted their response and ability to keep it small, and the visual and location data showed them the significance of the threat,” Kastner said. “They were able to dispatch higher-value resources quicker because they had high confidence on the threat.”
The fire was contained at 23 acres with no loss of life or structure.
The wildfire-detection space has also mustered resources from larger companies.
Christopher Van Arsdale, lead researcher at Google Research’s Climate & Energy group, leads the FireSat project, which plans to launch a constellation of fire-detection satellites early next year. A partnership among Google, the Earth Fire Alliance, and the Moore Foundation, the constellation will return high-resolution images of Earth every 20 minutes. With AI, the system will be able to detect fires about 5 meters by 5 meters in size, or roughly 1/400th the size of what current early detection satellites are capable of.
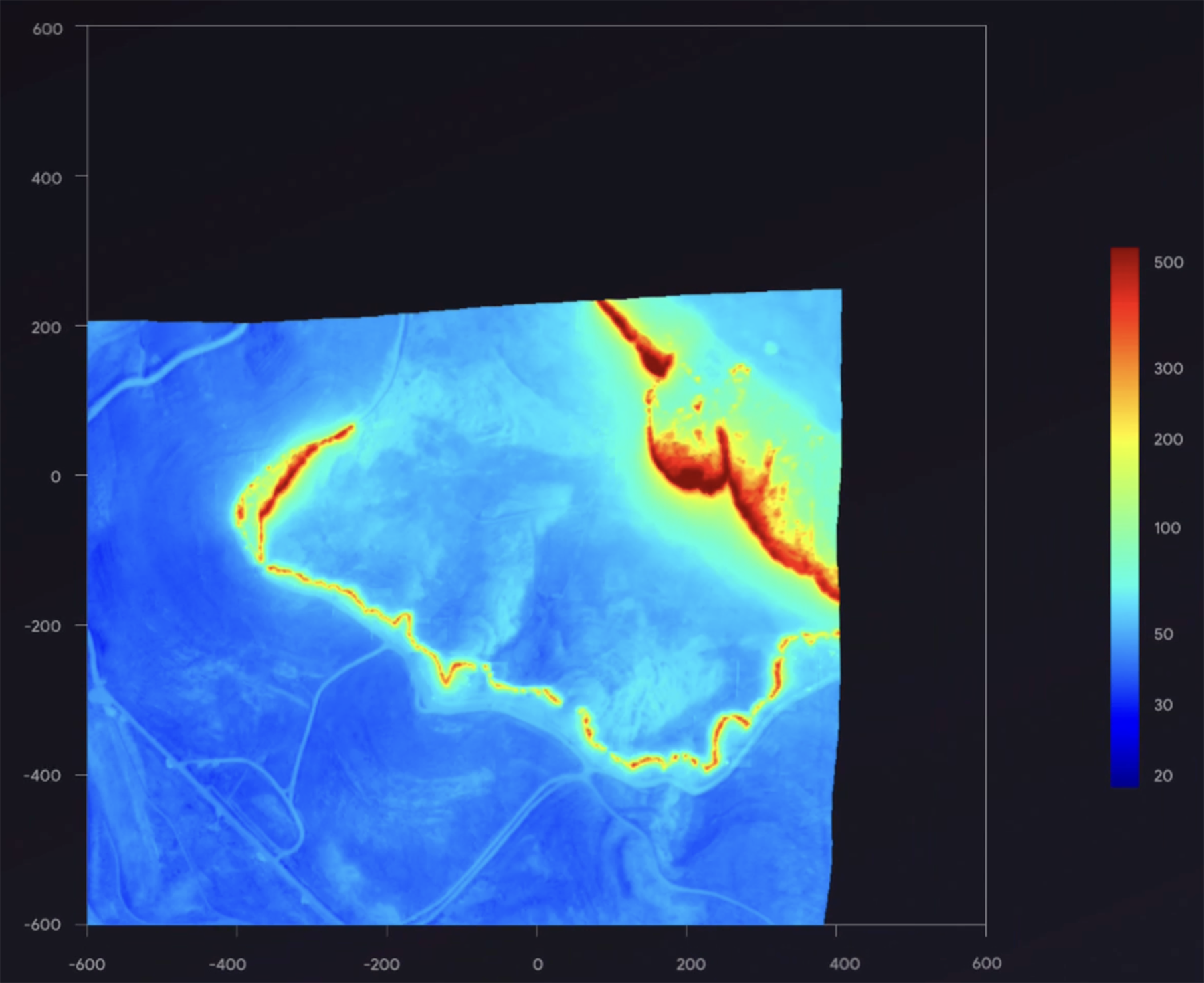
“One of the main hopes that I had when I started this project was essentially being able to get ahead of climate change for once and sort of giving ourselves the tools to help manage fires and especially ecosystems that are not well adapted for fire like the Canadian boreal forest,” Van Arsdale said.
Kastner also sees the new breed of detection systems as a working solution to growing wildfires.
“We don’t have to solve climate change to tackle the wildfire crisis,” Kastner said. “The use of technologies like Pano for early detection represents a pivotal advancement in our ability to manage and mitigate the impacts of wildfires today.”
Improved propagation models
Beyond greater coverage, more cameras provide more data on which to train the AI systems.
“From the evolution of the fires, you can infer back what the fire conditions were for the fire; you can see the fire rate of power, you can see how fast it burns,” Van Arsdale said. “You can go back and you can adjust your models to make sure that the ground truth that we collect from the FireSat satellites match the predictions that we’re making at the time for fuel load or for moisture content.”
With better models, the team can, for example, provide cities with a heat map of where fires are likely to start in the immediate future.
“You can make these (machine learning) models for propagation, or you can make better risk maps,” Van Arsdale said. “You understand in simulation, ‘OK, if I put a firebreak on the east side of town, is it beneficial or not?’ It’s a hard question to answer right now because we don’t have great tools for doing simulated fires, and we don’t necessarily have great fuel maps, and we certainly don’t have great propagation models.”
Kuester says that the vegetation types, biomass, and carbon metrics provided by these newer models can also inform other decisions, such as the value proposition of carbon sequestration in forests.
“Once you have a comprehensive model as part of our digital twin for California, we can actually use it to establish some biometrics and establish a better understanding and appreciation of what risk scenarios we look at,” Kuester said. “My challenge to our students is go ahead and tell me how many trees there are in California, literally.”
False positives
The detection systems are generally tuned conservatively, generating far more false positives than false negatives.
“We’re a little bit overcautious initially,” Kuester said. “We’d rather not miss anything which can develop into an extreme event. … (But) if you’re crying wolf too much, of course, a system would lose its value.”
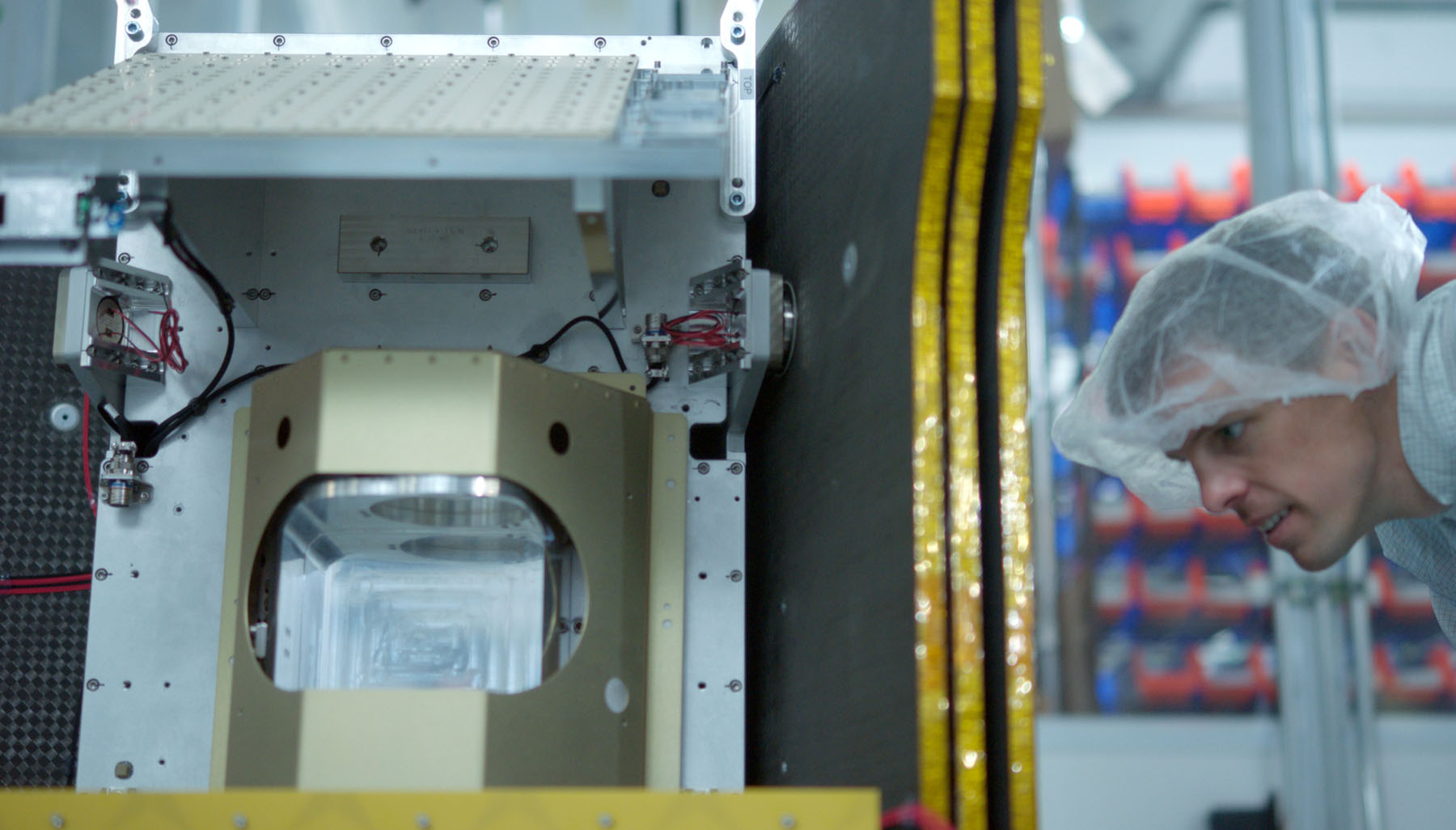
Similarly, Van Arsdale says agencies have asked for anything from every detected anomaly to a false positive rate under 10%. FireSat will generally aim to keep its false-positive rate under 50%, he continues.
Kuester hopes ALERTCalifornia will extend to other natural disasters. Alongside fires, floods and hurricanes will increase as climate change progresses, and he sees early detection as a key step to meeting those changes. He is heartened by what wildfire detection has accomplished already.
“The greatest success story of ALERTCalifornia are the fires that you’ve never heard about,” Kuester said. “They were detected, they were characterized, and they were responded to – put out – before a 911 call even came in.”