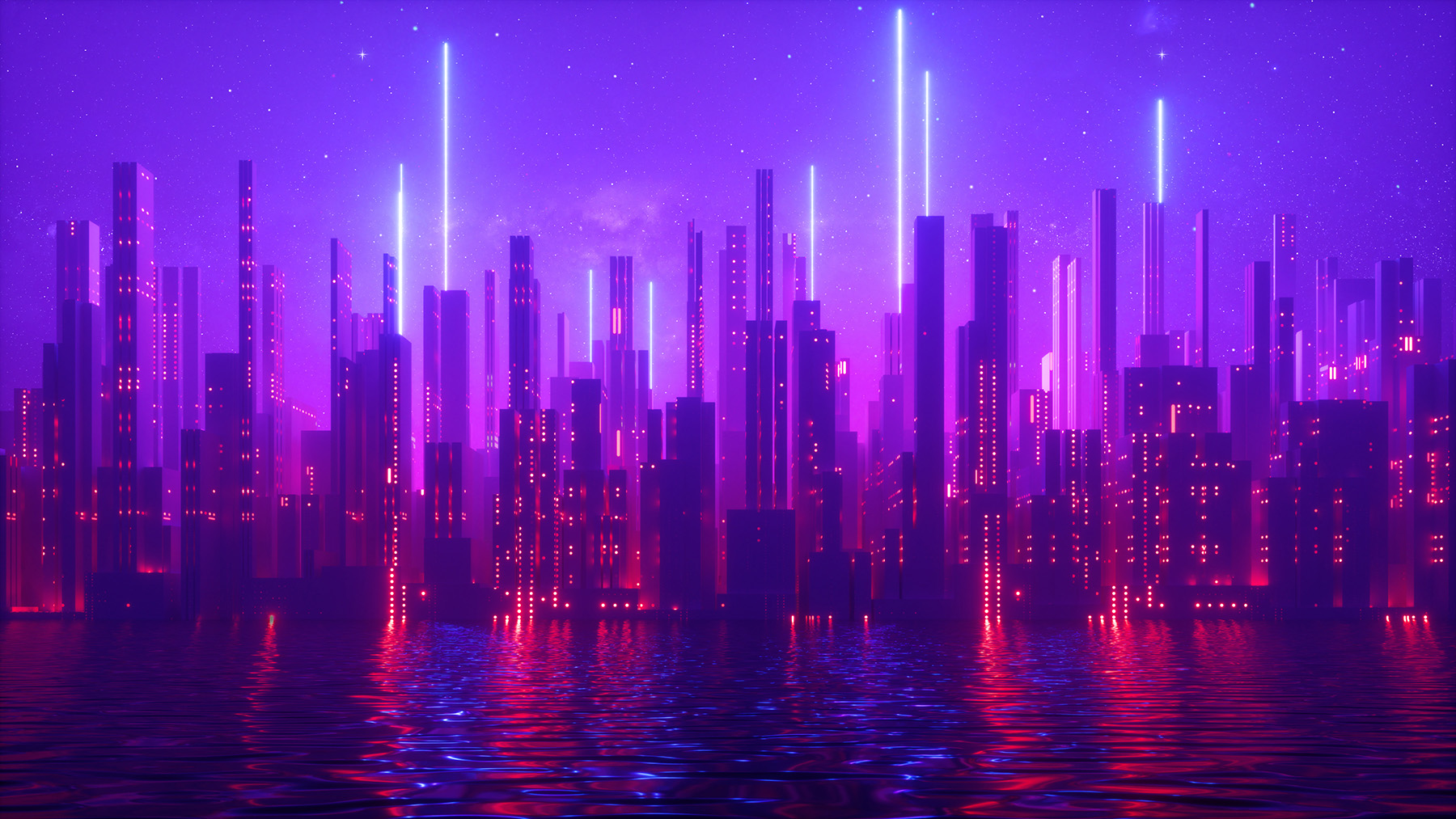
By Jeff Albee
Artificial intelligence offers civil engineers new tools for predicting flood damage. AI-generated flood models can rapidly consider multiple scenarios developed from enormous volumes of data.
For civil engineers, technology is a constantly evolving tool. Over the centuries, engineers have been at the forefront of solving problems through algebra, geometry, logarithmic tables, slide rules, calculators, computers, computer-based models ... and now, artificial intelligence.
Like any tool, AI has its place. Stantec is applying AI to computer-based models, especially for modeling floods.
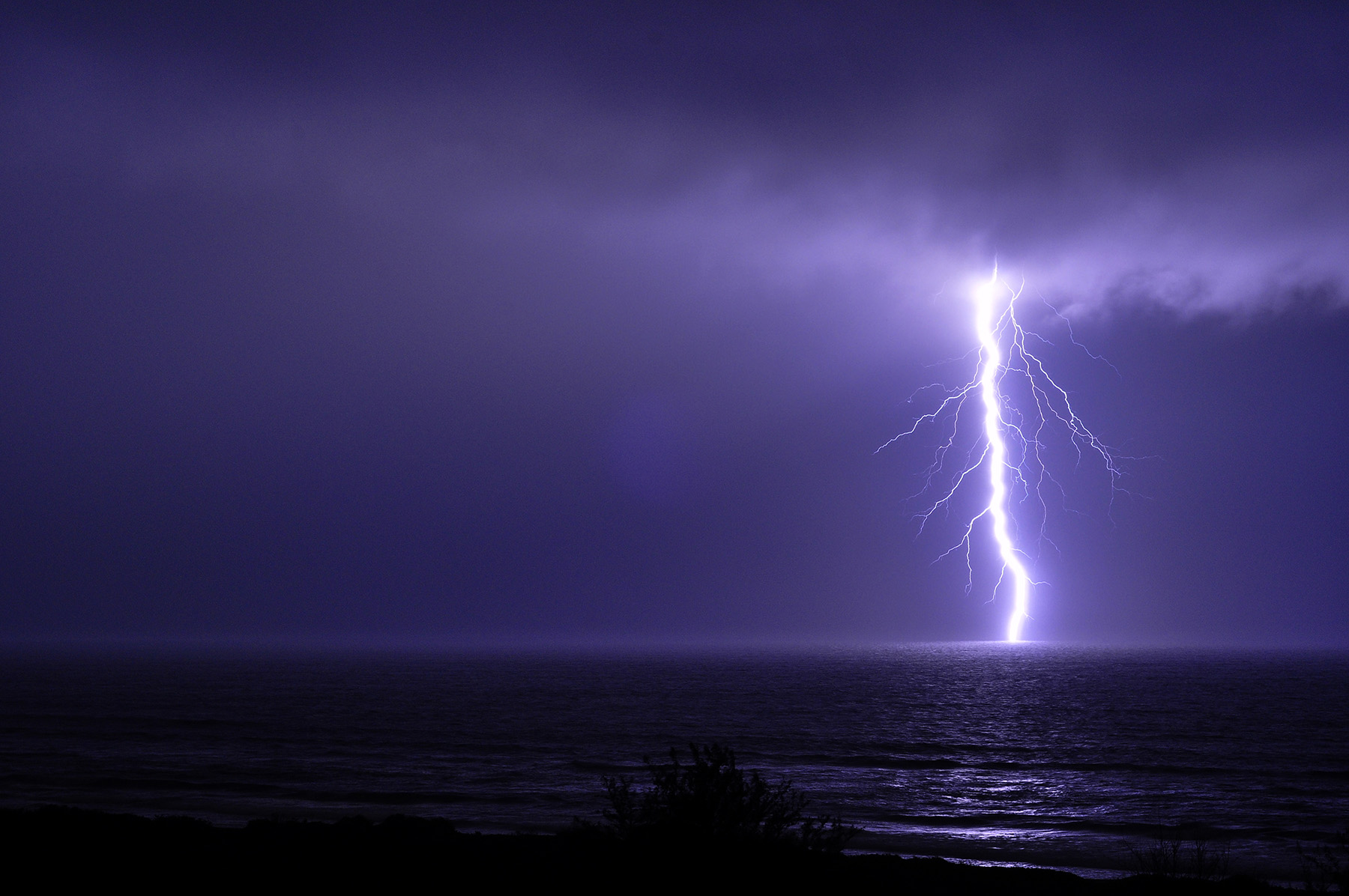
Floods are among the most devastating natural disasters, sweeping away houses, bridges, and other structures. They regularly destroy lives, livelihoods, crops, and transportation infrastructure. And with the changing climate threatening not only more storms but also more severe storms, floods are becoming a more pressing problem. Every month seems to bring a new “atmospheric river” or typhoon to some part of the world, with recent floods hitting hard in the southeastern United States, the Middle East, and southern Brazil, for example.
Improving flood projections
Flood analysis and mitigation are longstanding responsibilities of civil engineers, who work to redirect floodwaters away from vulnerable areas and reduce the effects of major inundations. To do so, civil engineers have often used on-the-ground surveys across watercourses and valleys or created simple projections for potential flood effects using the isolines on topographical maps to determine at-risk flood zones. More recently, computer-based modeling has enabled engineers to expand our understanding of how floods may impact an area. With computer models, it is possible to feed in a wide range of data about topography, vegetation, soil type, hard surfaces (e.g., roadways), and other factors that influence flooding.
Computer projections can factor in the impacts of various stormwater management measures — such as stormwater collection ponds and catchment basins, berms, and culverts — that enable engineers to formulate various storm scenarios and develop better remediation plans. But the huge volume of data being crunched by these computer models can present a problem.
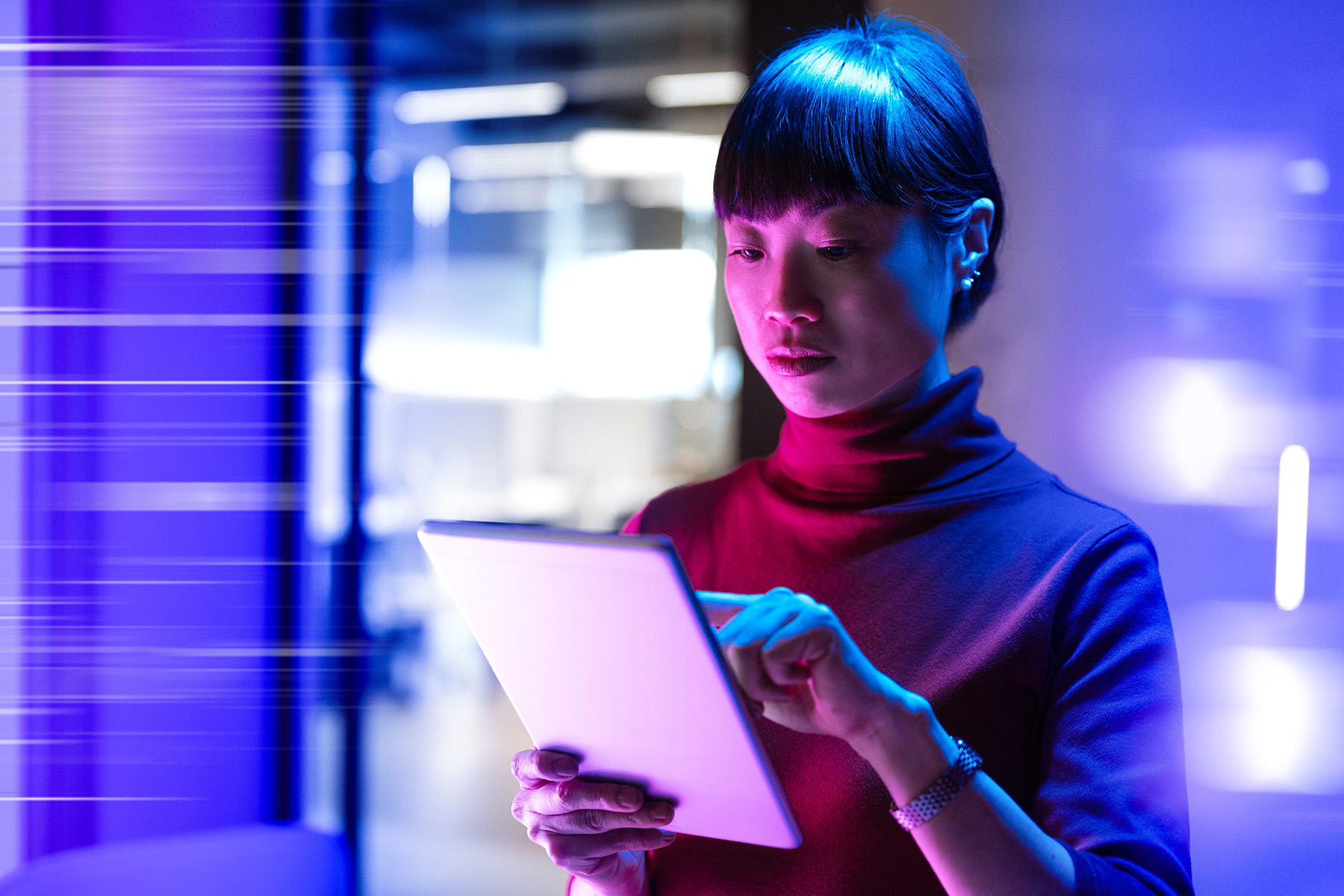
For example, if you want to model a 100-year storm, you begin by opening your modeling program of choice. You bring up the elevation model for the target area, the land-use model indicating pervious and impervious surfaces, the hydraulics and hydrology models, and perhaps other data including volume and duration of potential rainfall. You try to incorporate all those data and figure out the correlations, and you hit “calc.” And then you wait. And wait. If you are truly pulling together all the relevant variables, it could take hours, days, or even weeks to fully run the model.
And that is just for one scenario. The process must be repeated for any other level of storm. This is why many flood modeling programs can be compared to a powerful but slow truck rolling along.
Finding results faster
Although the current flood modeling programs are the gold standard for predicting floods, they would be more useful if they were faster. The pace of change as the result of climatic events calls for solutions that give users more access to computer power and model sensitivity but with greater responsiveness and speed. It is the equivalent of making that truck handle and accelerate like a sports car.
A powerful and fast computer-modeling solution can provide information that is useful throughout severe weather events, including:
- Before the event: Being able to project the impact of a storm helps engineers design safer communities. Many cities use scientific research on flooding to keep dwellings out of flood-prone watercourse valleys, prioritizing those spaces as parkland instead. Good modeling can support planning and preparedness and help determine areas that are likely to be impacted and to what extent. Some models can even help predict the depth, velocity, and water surface elevation of flooding, as well as its probability, and support what-if scenario planning.
- During the event: In the lead-up to and during the early stages of a flood event, models can support reliable and effective emergency response, guide evacuation efforts, indicate emergency routes, provide warning of high-risk areas, support road closure planning, and provide infrastructure protection planning.
- After the event: In the aftermath of a flood event, advanced modeling can efficiently provide insights about what occurred from both flooding and flood damage perspectives. This can inform response and rebuilding efforts and support updates to building codes and practices to mitigate impacts of future flooding. Good analyses can also help update the design of vast networks of storm sewers, catchment basins, stormwater ponds, dams, levees, and other infrastructure to alleviate the effects of future flooding.
Time to try AI?
When traditional computer-based models are not sufficient, engineers can turn to new data-driven simulations powered by AI. These AI simulations provide the ability to test a variety of scenarios, such as varying the volume of rainfall runoff to create the probability of flash and riverine floods and adjust their extent and depth. AI models are also being developed to help predict the damage from offshore storms that can produce extreme rain events and storm surges.
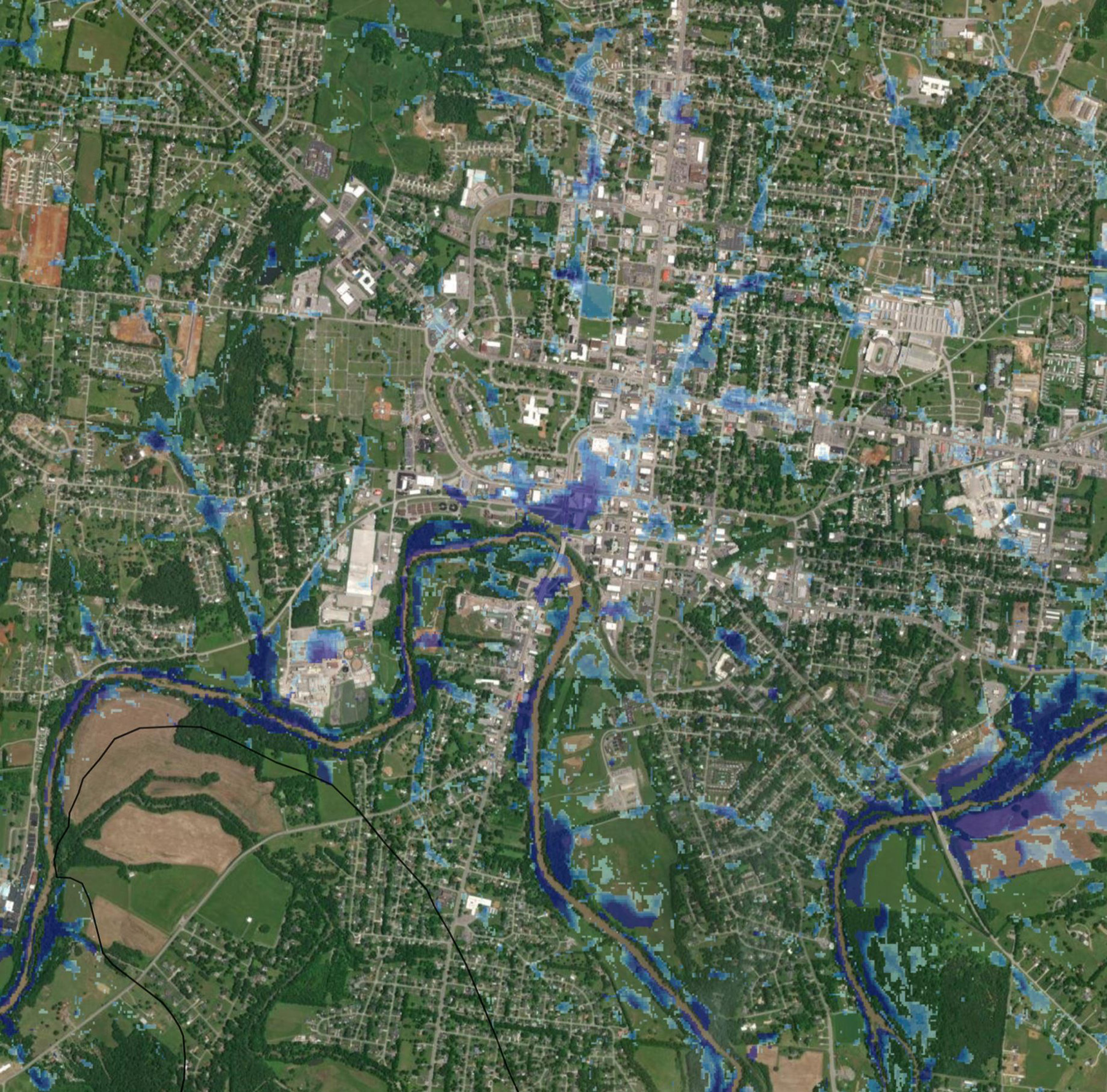
AI models rely on machine learning to incorporate the wisdom from past and current flood modeling scenarios. Such models can use the most representative scenarios for a given geography and create in a matter of minutes probabilistic results, consisting of thousands of runoff value scenarios. This provides a degree of efficiency and flexibility far greater than anything previously available to engineers.
However, to realize the full power of AI-enabled modeling, engineers must create high-quality, traditional, physics-based flood models to train the data-driven models on, letting the power of machine learning identify correlations and patterns for future predictions and measure the results. This is how flood modeling can achieve the reliability and speed needed for the before, during, and after scenarios mentioned earlier.
Filling the gaps
Boosted by machine learning models, engineers can fill in the data gaps so that their flood maps are more detailed and accurate. This will help them more easily determine which flood mitigation steps are needed — such as stormwater retention ponds, dams, levees, rain gardens, or green roofs — to manage a specific storm effectively. Worried city emergency managers can also plug in the parameters of forecasted or envisioned rain events to determine the likely extent of flooding. This will enable them to better alert their emergency response teams to close a particular road or notify residents of a potential evacuation order.
Perhaps most importantly, AI and machine learning allow engineers and others to more accurately model pluvial, or flash, flooding. These are floods generated by sudden intense rainstorms that inundate areas not normally affected by fluvial, or watercourse-based, flooding. The modeling of pluvial flooding is highly complex and requires a granular understanding of an area’s topography and hydrology. That means there is a huge amount of data to process and an enormous number of scenarios to examine, making it a task that AI and machine learning are particularly well suited to handle.
The appropriate and responsible use of AI and machine learning was key to the development of Stantec’s suite of flood management products. (For a case study, see the sidebar “Modeling Flood Risks in Tennessee”).
Getting the best results
To get the best results from data-driven models, engineers should avoid over-reliance on the technology. Without adequate understanding of the theories that the model is working from, it is easy to be either overly cautious or overly confident in a model’s predictions. This is where classical engineering skills are essential.
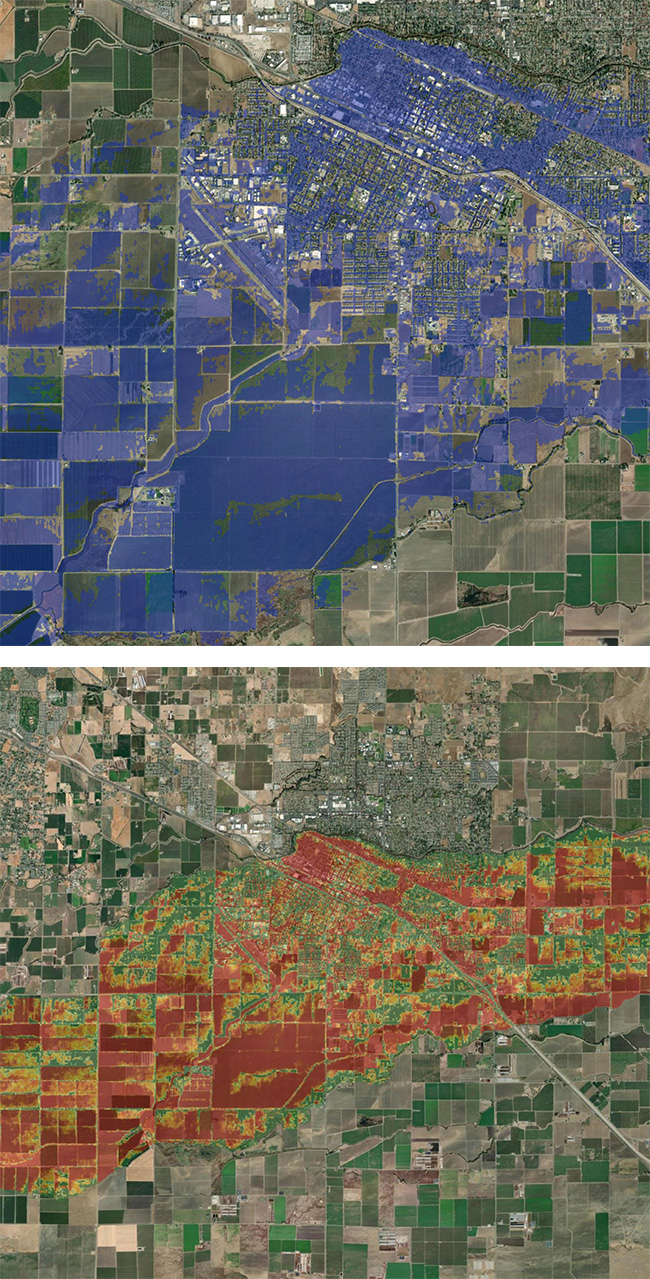
The use of sound engineering theory is what can keep a bridge from failing even when it has been hit by a flood-dislodged tree. That is why many design projects still require an engineer’s stamp indicating that a trained professional has either completed or checked the documents and found them to meet requirements.
But many AI and machine learning models are developed and trained by people with a highly specific set of skills. They understand software engineering, for instance, but do not necessarily understand the nuts-and-bolts world of civil engineering, including the range and nature of possible flood mitigation measures.
As a result, they do not always recognize when a conclusion made by the model is off base or when the design is unbuildable. That is why engineers with design experience, applying solid engineering theory, are indispensable in evaluating an AI platform’s output.
A trained and experienced engineer is also more likely to detect suspected AI inaccuracies and know how to deal with them.
The highest-quality results also depend on high-quality training data for the geography in question. Using Arizona model input to understand flood mitigation planning for North Carolina will produce a lower-quality result. The best models should provide transparency and replaceability of their training data.
Looking behind the curtain
Remember also that AI models should not be black boxes, generating results that were achieved through some unknown means. AI learns to recognize patterns by being trained on data — but if those data are of questionable provenance and quality, it may be a case of “garbage in, garbage out.”
Therefore, users should be ready to look behind the curtain to understand how the models were designed and trained.
Engineers can use their experience on similar projects to understand the steps that must be performed and then check to see that those aspects are covered in the computer-based model’s responses.
Across the industry, it should be common practice to disclose methods and deliver model metrics and methods as part of any science or engineering deliverable.
Before using any data-driven tool to predict an event as life-threatening as a flood, engineers need to understand the methods and metrics used to design the model in the first place. This implies a need for a consistent set of metrics for AI and machine learning models. Without consistent metrics, individual engineers cannot compare the results from various models, nor can two or more engineering teams work together.
This need for standardized, apples-to-apples ways of evaluating the AI system’s outputs is also essential because of the tendency of some AI tools to generate inaccurate or misleading responses in certain situations. If such faulty responses — known in the tech industry as hallucinations — are not spotted and culled, all the AI model’s results can be suspect. One solution is to measure the model’s performance against real engineering outputs. Training the model with data on actual floods will help it produce increasingly accurate results.
In the recent past, engineers who had been trained in ink-on-paper drafting had to learn computer-based drafting technologies. The same is true today and going forward. We live and work in a world in which AI is already making fundamental changes to the ways engineers design and deliver projects. This means that learning how to use these tools, learning to become comfortable with AI, is a key career skill for civil engineers to master.
SIDEBAR
Modeling flood risks in Tennessee
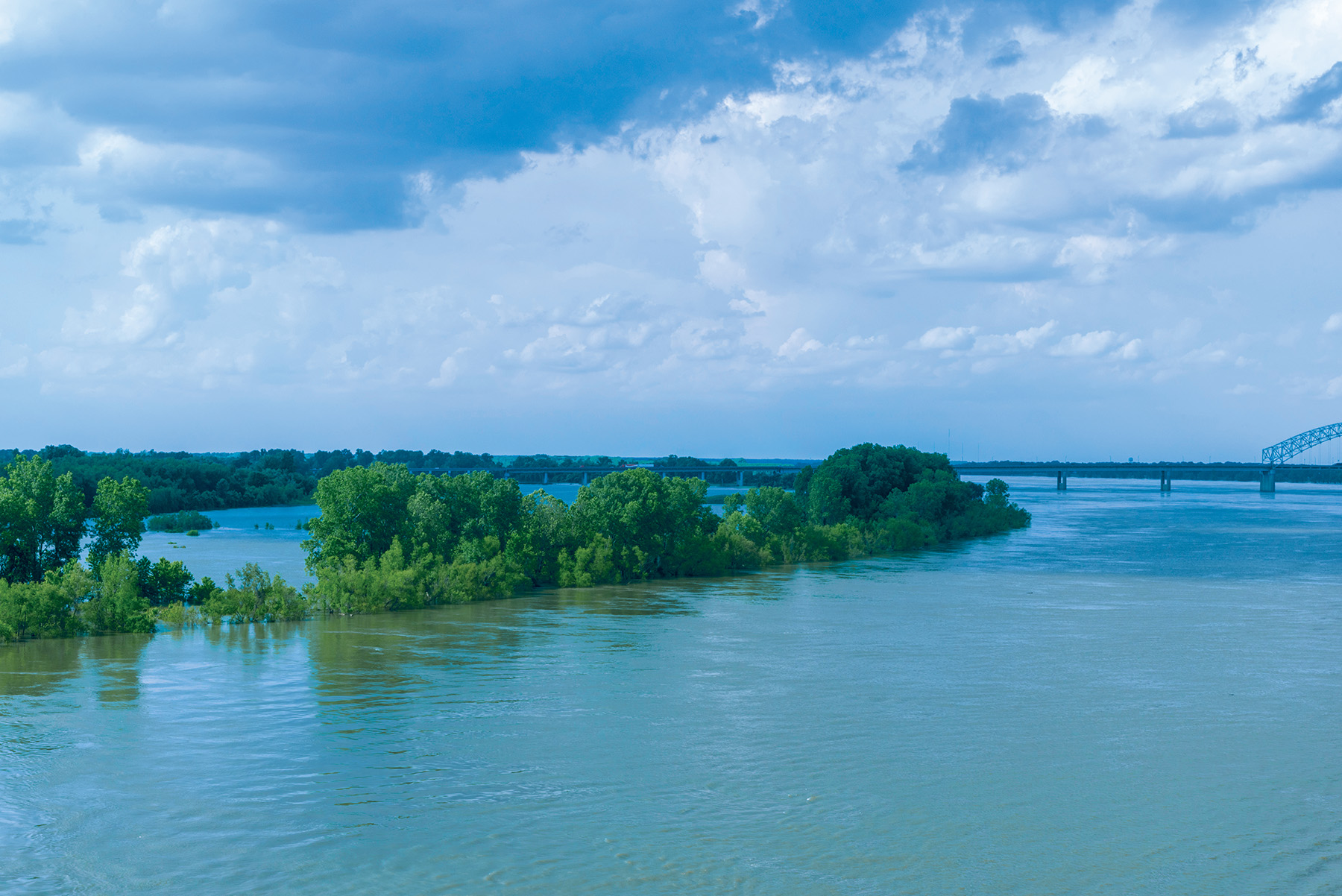
Twenty people lost their lives to flooding in the town of Waverly, in western Middle Tennessee, on Aug. 21, 2021. According to news reports, more than 270 homes were destroyed in flooding caused by heavy rains that inundated the region with as much as 15 in. of rain over a six-hour period.
This type of flooding is becoming more of a threat globally. The reasons include at least two key factors.
One is the increased frequency and intensity of storms, which means that stormwater is more likely to overwhelm storm sewers and overflow riverbanks. Second is the faster runoff of the rainwater from such storms because of an increase in hard surfaces like rooftops, roads, and parking lots, especially in regions with fast-growing populations, such as in Tennessee. These hard surfaces cause water to flow more quickly rather than being absorbed by the soil, which increases the danger of flooding.
To help mitigate future tragedies in Tennessee, the flood modeling team in Stantec’s Nashville office looked for ways the company’s Flood Predictor model could have helped in the case of the Waverly flood. Working proactively, the team fed data about the weather conditions and topography of the area into the artificial intelligence-powered model and compared the model’s results to the actual outcomes on the ground.
The results demonstrated a very high correlation between what happened on the ground and what the model predicted, specifically related to the extent of the flash floods and the depth of flooding in the impacted areas. When the team shared these findings with the Tennessee Department of Economic and Community Development, it commissioned a pilot project to test the concept through fiscal year 2024. This involved integrating Flood Predictor into the state’s online repository of resilience data for six counties in western Tennessee, enabling community leaders and emergency departments to quickly access flood risk insights before, during, and after severe weather events.
The drumbeat of severe weather events continues to put more communities at risk. When it comes to the dangers posed by flooding, civil authorities can help keep up with the pace of change and level the playing field by turning to software tools and platforms powered by AI and machine learning.
Jeff Albee is the vice president and director of digital solutions for Stantec. He is based in Nashville.
This article first appeared in the November/December 2024 issue of Civil Engineering as “AI for Flood Modeling.”