By Sarah Derouin, Ph.D.
The potentially dire effects of rumbling, underwater earthquakes are constant threats to many coastal communities. Even earthquakes that occur far from shore can produce deadly tsunamis that can wreck seaside cities, devastating buildings and killing thousands.
The risk of a tsunami forming is related to the characteristics of underwater earthquakes and how their displacement ripples outward. Collecting and analyzing information about earthquakes — from land or sea sensors — in real time is the key to calculating tsunami risks. These data are used for early warning systems that are crucial for coastal areas at risk of waves.
New research in Physics of Fluids shows the possibility of getting real-time earthquake information from underwater sensors to add more information to risk calculations. The researchers tested how hydrophones can pick up the unique acoustic radiation — that is, sound — from earthquakes. From these data, the team used artificial-intelligence-informed models to parse out the earthquake characteristics — including fault shape and slip type, uplift speed, and duration of shaking — which influence the size of tsunamis.
The researchers see their approach as another tool in tsunami forecasting and risk mitigation for coastal regions.
Careful monitoring
Although tsunamis can be triggered by landslides and volcanic eruptions, the vast majority of large waves are triggered by underwater faults that displace the water above. When the ocean crust suddenly ruptures, a rippling wave can spread across oceans, inundating coastlines thousands of kilometers away.
“Tsunamis can be highly destructive, causing huge loss of lives and devastating coastal areas,” says Bernabe Gomez, Ph.D. and co-author of the new paper. He notes that understanding the nature of an earthquake is crucial for assessing the potential and characteristics of a tsunami. It is also important in order to issue early warnings and give ample time for evacuations.
Currently, forecasting tsunami risks is an iterative process that takes place over minutes to hours. “What we typically have to do is put out a forecast within five minutes based on seismic information alone. Then as we get more information and more sensors start to report, we're continually updating that forecast and making it more accurate,” explains Christopher Moore, the deputy director of the National Oceanic and Atmospheric Administration’s Center for Tsunami Research. (Moore was not involved in the research.)
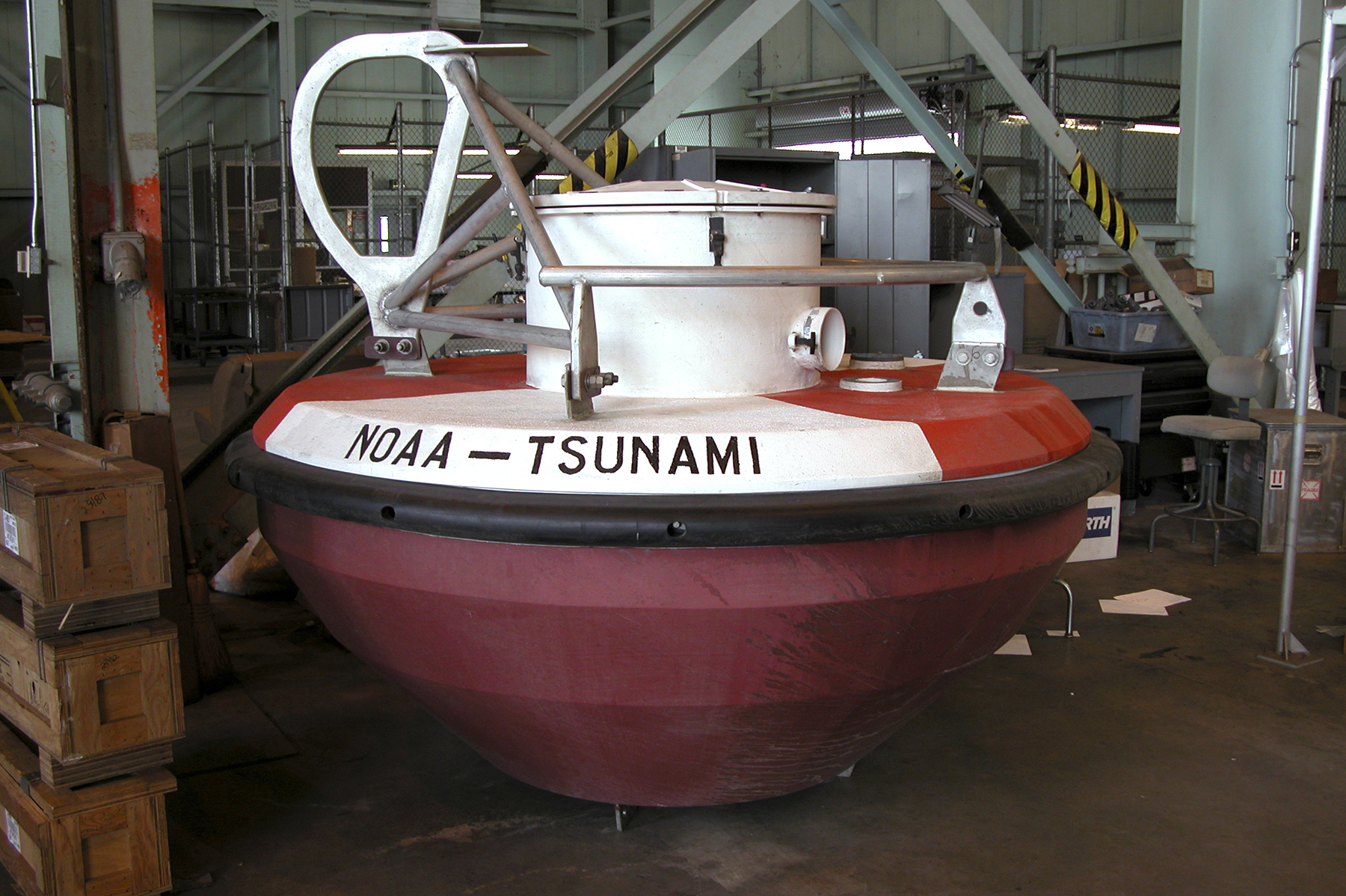
Sensors can be on land (seismometers), circling the globe (global navigation satellite system), or on the ocean (Deep-Ocean Assessment and Reporting of Tsunamis buoys). The DART buoy array is a set of floating sensors placed around the world but is heavily concentrated within the seismically active Pacific Ocean region.
Moore explains that while DART gives highly accurate information, the sensors are expensive to place and maintain and as such are only placed in the most critical regions. “We've done our best to optimize DART buoy placement, according to not only where earthquakes occur, but where people live,” he says. But the distance of the buoys from some earthquake epicenters means there can be a lag in new information of 10-90 minutes after an earthquake.
“We're continually trying to find new sensors to slot in between the time where we have seismic information and when we have that highly accurate DART buoy information,” says Moore. This can include GNSS information, disruptions in communication cables, or even hydrophones.
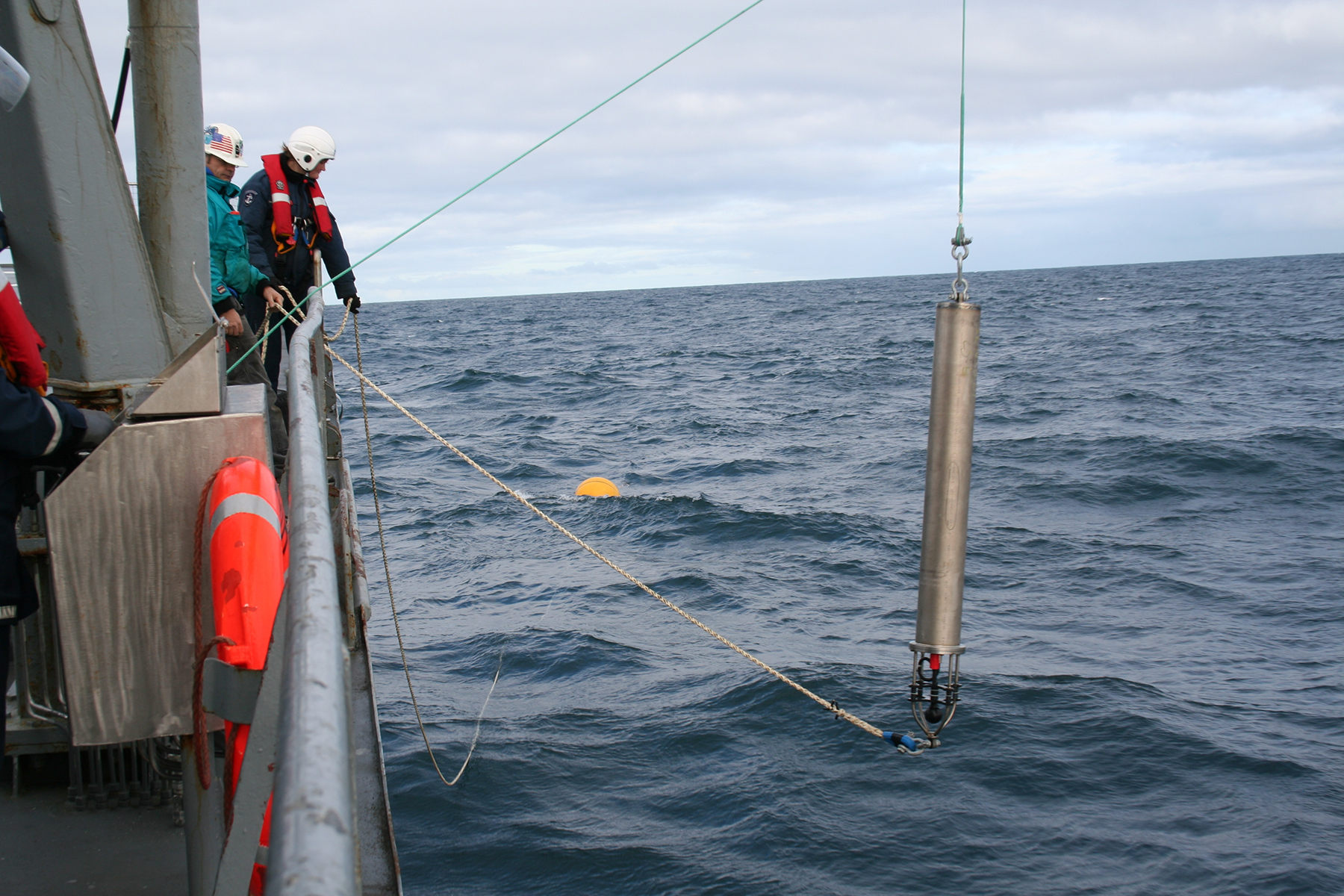
Hydrophones are placed on the ocean floor and can detect sounds from all sorts of activities, from underwater eruptions to bomb testing to earthquakes. These sound waves, also called acoustic-gravity waves, travel at the speed of sound (1,500 m per second in seawater) and can often play off the ocean floor, doubling their speed.
“Sound carries information about the originating source, and its pressure field can be recorded at distant locations, even thousands of kilometers away from the source,” says Gomez. “They carry information about the earthquake’s characteristics and can be recorded by hydrophones in the far field.”
Listening to earthquakes
Gomez and co-author Usama Kadri wanted to see if the sound signatures from different fault ruptures could be parsed out using machine learning algorithms. They looked at four tsunami scenarios that occurred within the Pacific Ocean. Their sample scenarios were limited by the availability of hydrophone recordings.
“From the available Comprehensive Nuclear-Test-Ban Treaty Organization database, which is managed by the International Monitoring System, we chose about 200 earthquakes in geographic locations with minimum noise,” says Gomez. They used those historical data to train the machine learning algorithms to classify the type and magnitude of earthquakes. This training allowed the team to back-calculate how acoustic waves could be used to present details of earthquakes’ characteristics, including size, uplift speed, and duration.
Gomez says the approach was successful in creating a description of an earthquake in almost real time with extremely low computational efforts. “However, there are still some discrepancies between the retrieved effective characteristics and the actual ones,” he says, adding that there are improvements that could be made to the modeling inputs. Gomez also notes that the “use of more data in the artificial intelligence training could decrease the associated uncertainties.”
“My first response is this is definitely a novel thing and a new technology,” says Yong Wei, Ph.D., ocean engineer researcher at the University of Washington and NOAA affiliate, who was not involved in the study. He adds that while the method is something to explore in the future, there should be more development of AI algorithms.
“When comparing their prediction with the DART measurements, you can see there are some discrepancies,” notes Wei. He says that these differences are likely because of how the researchers trained their model. “They mentioned they used about 201 events — maybe that's not enough. Usually, when you do AI, you need a lot of data to train your model. When you don't have enough data, then your model accuracy will drop.”
Moore says this research paper is trying to use an AI technique that could be used for the entire ocean. In contrast, he notes that NOAA Tsunami Research Center researchers have also been working on AI modeling in the Pacific Northwest. They have had some success, but he said it was because of a massive effort on a small area of coastline.
“It took the efforts of several seismologists to come up with a database of possible solutions, a data scientist and mathematician to train an AI model, Yong Wei to do modeling for all of those thousands of runs,” says Moore. “It's very promising results, and it's super exciting work — and it's just a drop in the bucket.”
Hydrophones and early warning
“Artificial intelligence can play a prominent role in the classification of earthquake types,” Gomez says. “In combination with state-of-the-art acoustic-gravity wave technology, we can have a more reliable real-time tsunami warning system.”
“This work is part of a larger project aiming at enhancing warning systems from natural hazards,” says Gomez. “The nature of the developed technology is complementary, and as such we look forward to collaborating and complementing experts on further enhancing the system.”
The method has the potential to be an innovative tool in tsunami forecasting, say Wei and Moore.
“We have hope that AI will lend itself to solve some of the problems we have in characterizing a tsunami generated from something like a landslide or a volcano,” says Moore. Because these natural events do not have associated earthquakes, he says it is harder to characterize the location and size of a tsunami that might be generated.
Hydrophones and AI modeling could be useful tools in the development of tsunami warning systems. “We all know that new technology needs time to evolve,” Wei says. “I think this technology has a great potential to be implemented in the future system.”
This article was published by Civil Engineering Online.