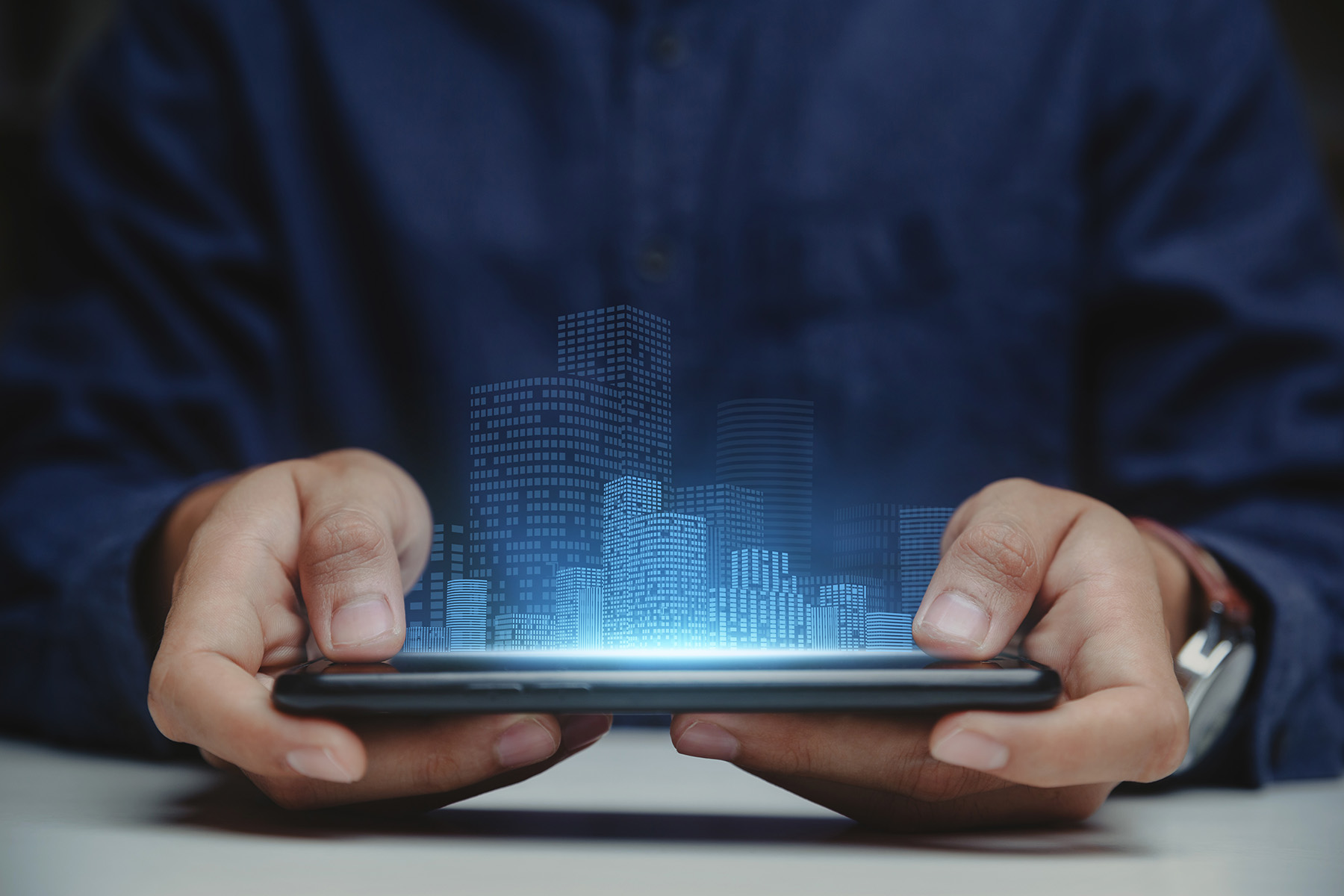
By Robert L. Reid
Artificial intelligence will likely change the civil engineering profession in profound ways. But in the end, leading civil engineers believe that greater use of AI will only increase the need for human involvement in designing, constructing, and maintaining the built environment.
What does artificial intelligence mean for civil engineers? The answers are as varied as the potential applications of the AI tools themselves. Elsewhere in this issue are case studies of how several engineering firms are using AI tools for asset management, transportation engineering, and creating flood models. This article, however, provides an overview of some critical issues and concerns common among engineers who use AI, plan to use it in the future, or are just thinking about this new technology.
AI generally involves the use of computers or other digital technologies to “replicate the function of the human brain, of human intelligence,” says David Odeh, P.E., S.E., F.SEI, F.ASCE, a senior vice president and national director for WSP USA Building Structures. “You see a lot of hype about AI in the media,” Odeh adds, “how computers will replace engineers, how it’s a revolutionary technology.”
And while Odeh does believe that AI will “fundamentally transform our industry,” he also feels that structural and other civil engineers are not about to be displaced by algorithms anytime soon. For most civil engineering firms, AI is still a fairly new technology that has “not effectively been applied yet to problems in a way that our engineers can use it on a day-to-day basis.”
So, while AI will likely change the civil engineering profession dramatically, “we have a lot more work to do to leverage AI in our profession,” Odeh says.
Other engineers, however, feel the age of AI is already here. AI is not something that is going to happen in the future, “it is happening now,” according to Stephanie Slocum, P.E., M.ASCE, the founder and CEO of Engineers Rising LLC. Participating in a February 15 ASCE webinar, “The Future of AI in Civil Engineering,” Slocum stressed that if a civil engineering firm has not started developing a policy around AI, “that needs to happen sooner rather than later.” Firms that are adopting the new technology will enjoy a competitive advantage in the marketplace, she explained.
Future past
While AI can sometimes seem like science fiction, the basic technology has been around for decades. Anand Stephen, P.E., C.M.E., M.ASCE, the digital delivery leader for the roadway business group of Gannett Fleming, recalls that one of his civil engineering graduate program professors at the University of Toledo used machine learning algorithms to conduct pavement analyses in the late 1990s. Machine learning is considered a subset of AI.
Likewise, David Martin, A.M.ASCE, a design engineer at Degenkolb, explained during the ASCE webinar on AI that the technology has been used in the civil engineering industry for at least the past 20 years. “This isn’t actually anything new,” Martin said during the webinar. But it was just within the past few years that AI tools became much more “approachable” and user-friendly for civil engineers, spurred on by the development of so-called large language models like ChatGPT, Martin says. LLMs produce text that sounds like a human conversation.
Such systems “totally democratized the utilization of AI,” adds Burcu Akinci, Ph.D., F.ASCE, head of the Department of Civil and Environmental Engineering at Carnegie Mellon University. With systems like ChatGPT, “you don’t need to understand coding to be able to ... take advantage of AI,” Akinci explains. “These systems have interfaces that let you talk with autonomous agents that gather information for you and generate answers to your prompts in the way that you prefer.”
To accommodate the growing interest in AI, Carnegie Mellon is launching an online graduate certificate program, AI Engineering — Digital Twins & Analytics, that the university’s course description says was designed for working engineers in senior and managerial roles who are looking to advance their careers by developing these leading-edge AI and digital twin skills and who are learning how to implement them in their workplaces.
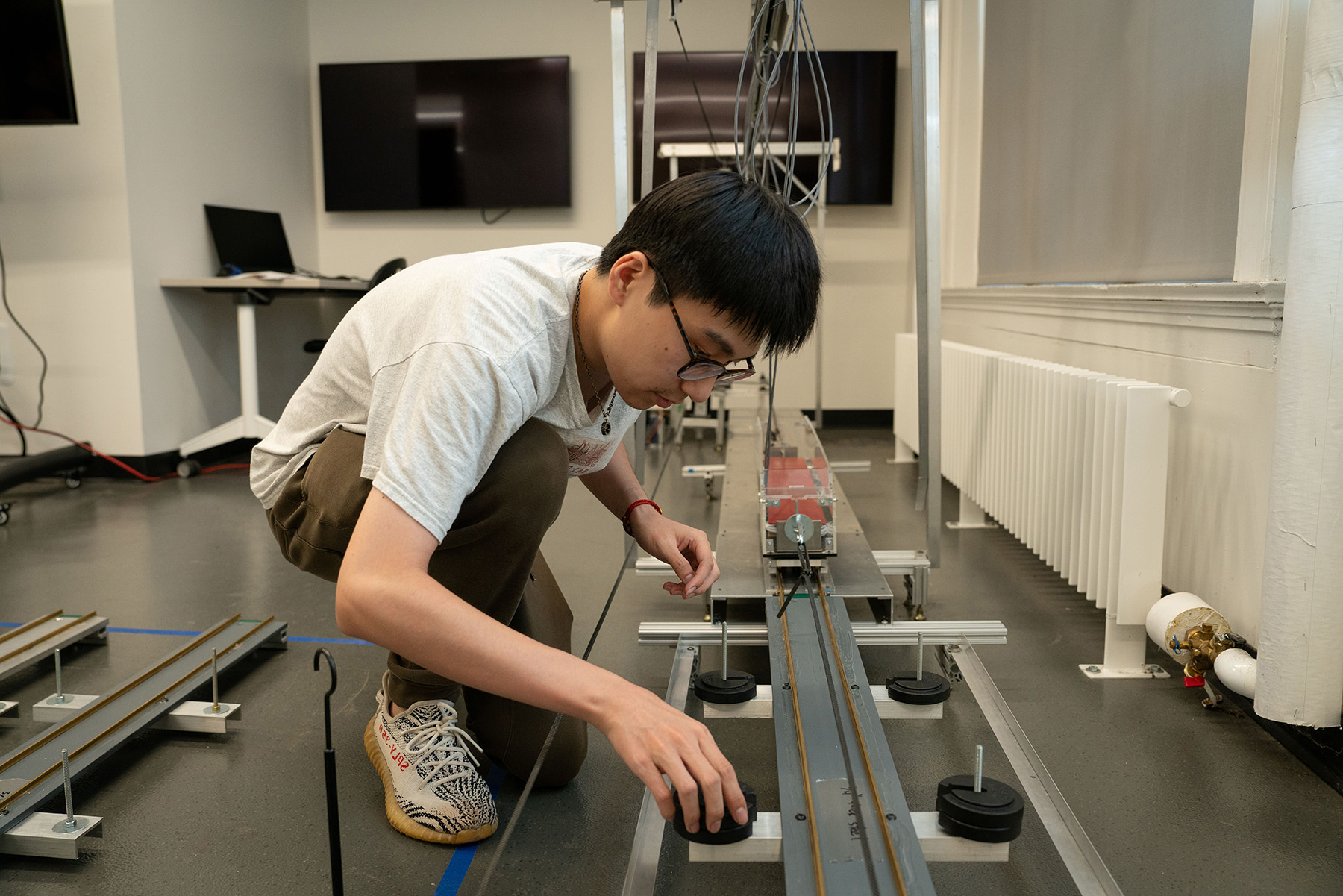
Although the certificate program will feature virtual coursework, there is also a 20 ft long physical model with a highly stylized “locomotive” that participants can interact with and collect data from remotely, Akinci notes. Located in a CMU laboratory, the physical model will provide a test bed in which the locomotive — represented by a clear rectangular box — is pulled back and forth across a bridge on tracks. Cameras will continuously stream the laboratory testbed to a portal on the class website so that students can log in and select the variables they wish to test — such as speed, locomotive load, bridge stiffness, and types of damage — and then run the test remotely. They can then watch the trial live, with all data collected during the test being packaged and sent to them.
The system is designed to reinforce the idea “that a central part of digital twins is the integration between the physical and virtual environments,” explains Katherine Flanigan, Ph.D., A.M.ASCE, an assistant professor of civil and environmental engineering at CMU.
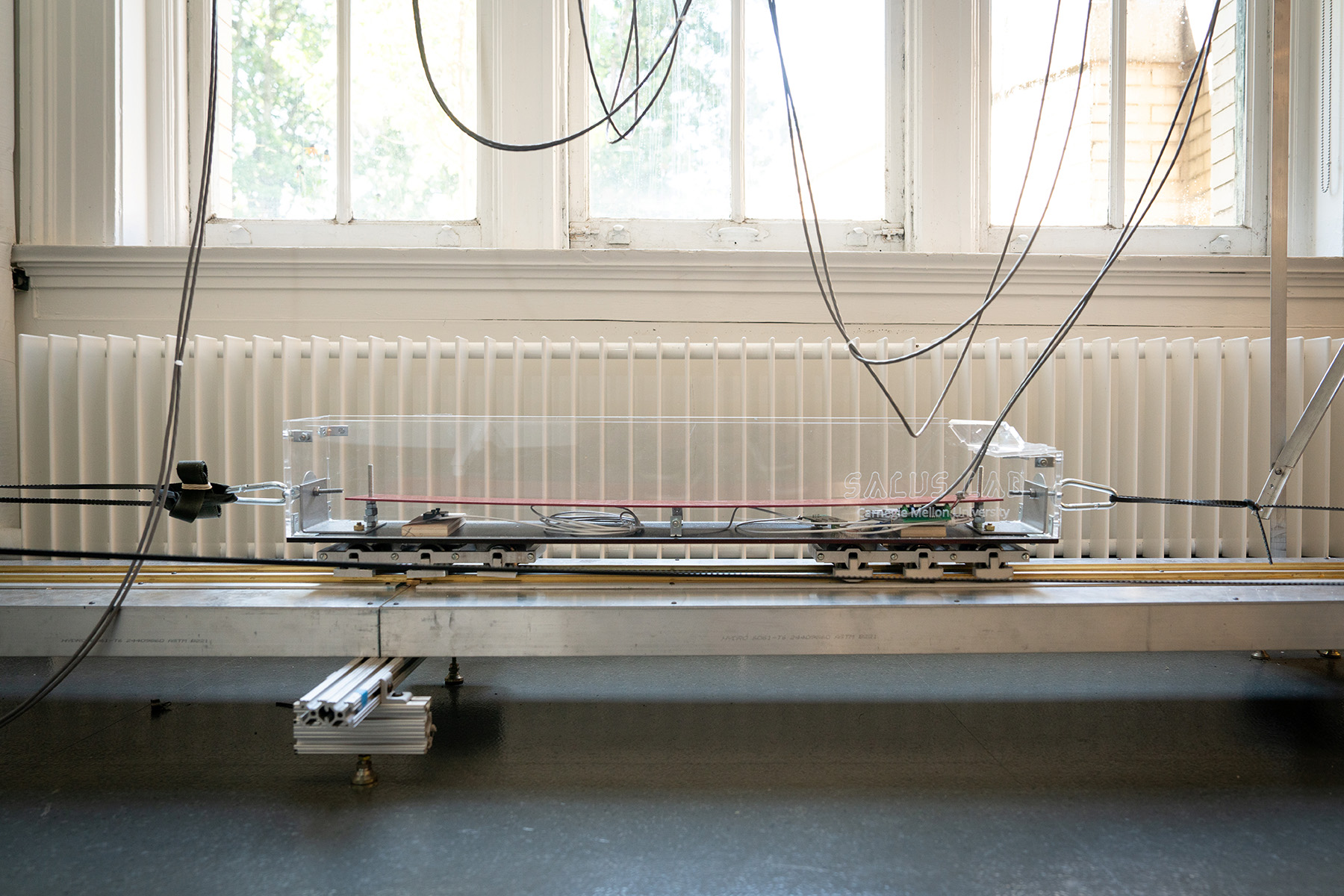
AI action
Artificial intelligence is potentially “a tool of no limit,” according to Dilip Barua, Ph.D., M.ASCE, a hydraulics and coastal engineer and consultant in Vancouver, British Columbia, Canada. Barua has written extensively about AI on his personal website, Wide Canvas, and he participated in ASCE’s AI webinar.
According to Barua, “AI routines and algorithms that can think almost like a civil engineering professional are in different stages of research and development.” These routines and algorithms “have the potential to enhance the capabilities of analysis and computation, streamlining performance, and helping with examining and screening the solutions of a particular problem.”
Barua stresses that adaptation is one of the most important characteristics of AI systems. “A normal computer program cannot adapt,” he notes. But the ability of AI systems to learn from training data, to use those data, and to change computational direction based on the queries or inputs received, makes AI systems “unique, smart, and powerful.”
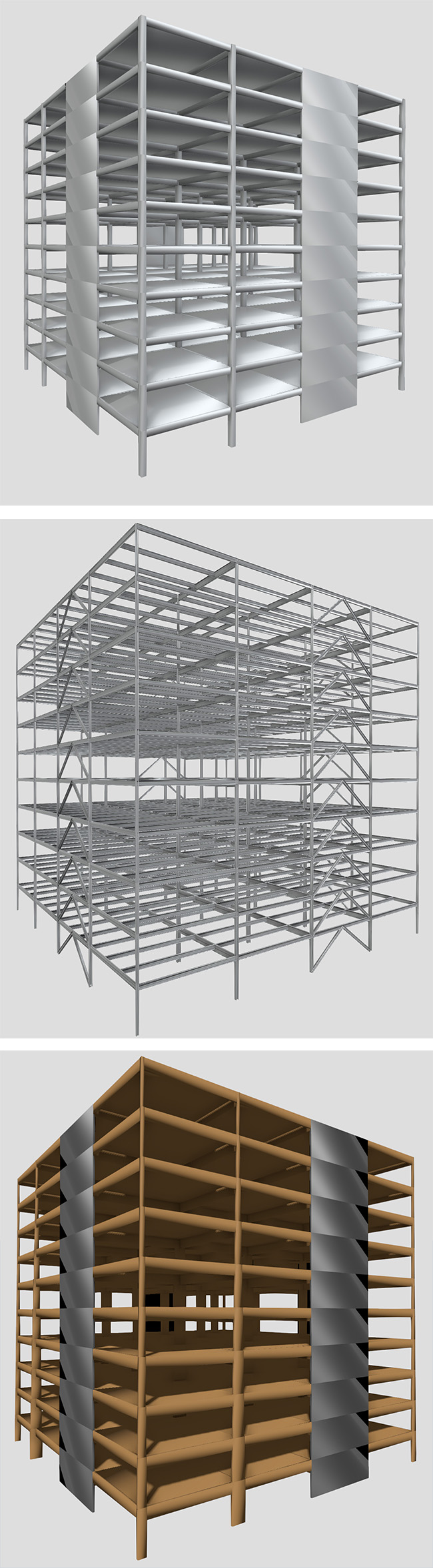
Civil engineers should start asking questions about how the training algorithms for AI systems can include the many tools, methods, models, principles, and standards that engineers use in their daily professional lives, Barua adds. He also believes that civil engineers in North America are not taking advantage of AI tools as much as their counterparts in Asia. Barua points to efforts like those at the University of Tokyo to reconstruct buildings “as digital twins in cyberspace, with ... an optimal control framework for energy systems ... developed by combining prediction models and optimization methods based on AI,” according to the university’s website.
Thornton Tomasetti is one U.S.-based engineering firm that has strongly embraced AI, notes Robert Otani, P.E., M.ASCE, the firm’s chief technology officer. In 2011, Thornton Tomasetti even established an in-house group, CORE studio, that has developed several artificial intelligence and machine learning technologies designed specifically for the architecture, engineering, and construction industries. CORE studio’s Asterisk app, for example, “generates designs at the schematic and concept level (in seconds) that would take months using conventional methods,” according to Thornton Tomasetti’s website.
Another CORE studio product, T2D2, is a cloud-based system using computer vision, a form of AI, to automatically detect “visible damage ... in concrete, steel, masonry, and timber structures,” explains the website. The system “uses recent advances in artificial intelligence to detect damage and defects by analyzing inspection images and video” based on “annotated datasets consisting of thousands of images taken during manual structural and facade inspections.”
In 2021, Thornton Tomasetti spun off T2D2 as a separate company.
At Building Structures, “we use AI tools in a variety of areas to improve our technical work and productivity,” notes Odeh. “We are looking at large datasets and trying to extract useful trends. For example, we generate large amounts of data from building analysis models ... on structural response to loads, the quantities and types of materials, and the interaction of systems,” Odeh explains.
“Our firm is exploring ways to use machine learning to extract useful results from that data, to see insights that might help our engineers design buildings better,” Odeh says. For example, the firm is identifying ways to reduce embodied carbon in a structure by examining similar buildings and developing insights from those earlier projects to help meet the sustainability goals for a new building.
Other uses include generating images for marketing material and using LLM systems to help engineers write technical reports and emails. “These uses of AI help to save time and increase the productivity of our professionals,” according to Odeh.
Datasets large and small
AI excels at providing “rapid brainstorming and idea generation” when working with large datasets, noted Slocum during the ASCE webinar. For instance, if someone wants 20 ideas on how to reduce carbon in a civil engineering project, the system “will give you 20 ideas instantly,” Slocum says. In the past, however, “you probably would have sat in a meeting with a number of people and ... come up with five ideas before you started picking apart why each one would or wouldn’t work.”
At the same time, AI can also help when the available data are limited, notes Sarah Billington, Ph.D., A.M.ASCE, chair of the Civil and Environmental Engineering Department at Stanford University and a senior fellow at the school’s Woods Institute for the Environment. Billington has worked with her colleague Dan Iancu, Ph.D., an associate professor of operations, information, and technology at the Stanford Graduate School of Business, on a project that applies AI to predict the use of forced labor in the supply chains for construction materials and in agricultural industries such as cocoa farming in Africa. In the cocoa study, researchers are building predictive models of child labor in Ghana using a small dataset that has extensive, reliable data as well as a countrywide dataset that is rather sparse, she notes.
AI tools help “leverage the data we have when we don’t have much,” Billington says, explaining that the smaller, more detailed datasets can be used to help train the AI system to make predictions in larger settings.
This approach can be applied to the construction material supply chain challenge as well. One of Billington’s colleagues, Rishee Jain, Ph.D., A.M.ASCE, an associate professor of civil and environmental engineering at Stanford, is likewise using AI to conduct “no touch” energy audits of thousands of school buildings across California.
Jain is using an integrated simulation and machine learning approach to overcome data sparsity challenges and pinpoint potential energy efficiency retrofits without setting foot in the building, Billington explains.
Irreplaceable engineers
A need for human involvement is another critical aspect of civil engineering’s interaction with artificial intelligence. “There is no replacement for qualified expert opinion and the knowledge of qualified human engineers,” Odeh says. Engineers need to combine AI tools with their professional judgment, knowledge, experience, and even intuition, Odeh says.
In the ASCE webinar, Slocum outlined a potential problem regarding the use of AI for writing technical reports. A civil engineer can go on a site visit and, using a “conversational AI” tool and the necessary prompts, generate a draft report in less than five minutes — a task that normally takes hours to complete, Slocum said. But the engineer must understand the capabilities and limitations of the technology and gain “the expertise to check what the output is.”
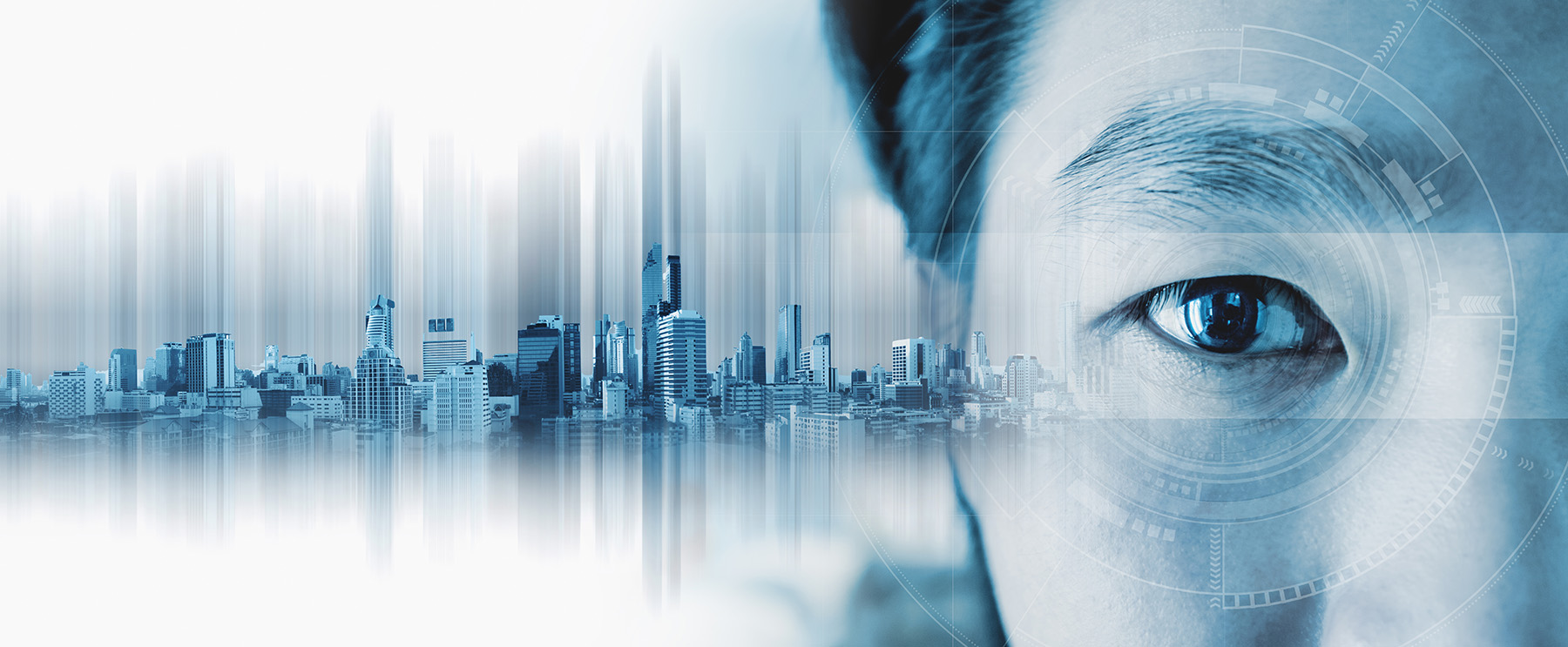
For example, if engineers enter technical terms into a natural language system like ChatGPT and ask it to define those words, they may discover that “the output sounds really good, it sounds like it knows exactly what it’s doing,” Slocum explained. But the outputs can also be “really, really wrong!” Slocum stresses.
AI tools, especially LLMs, are subject to a problem called “hallucinating,” which occurs when a person asks the system a question it does not know the answer to, says Otani. “So, it makes something up.”
To protect against AI hallucinations, Thornton Tomasetti instituted a policy that requires employees to use non-AI sources to check the responses from AI systems. “The biggest fear is that someone uses AI directly without checking, and the information is completely made up,” Otani explains. This verification process can involve such actions as performing separate calculations to confirm the results from the AI system or finding a document that corroborates the AI response, Otani says.
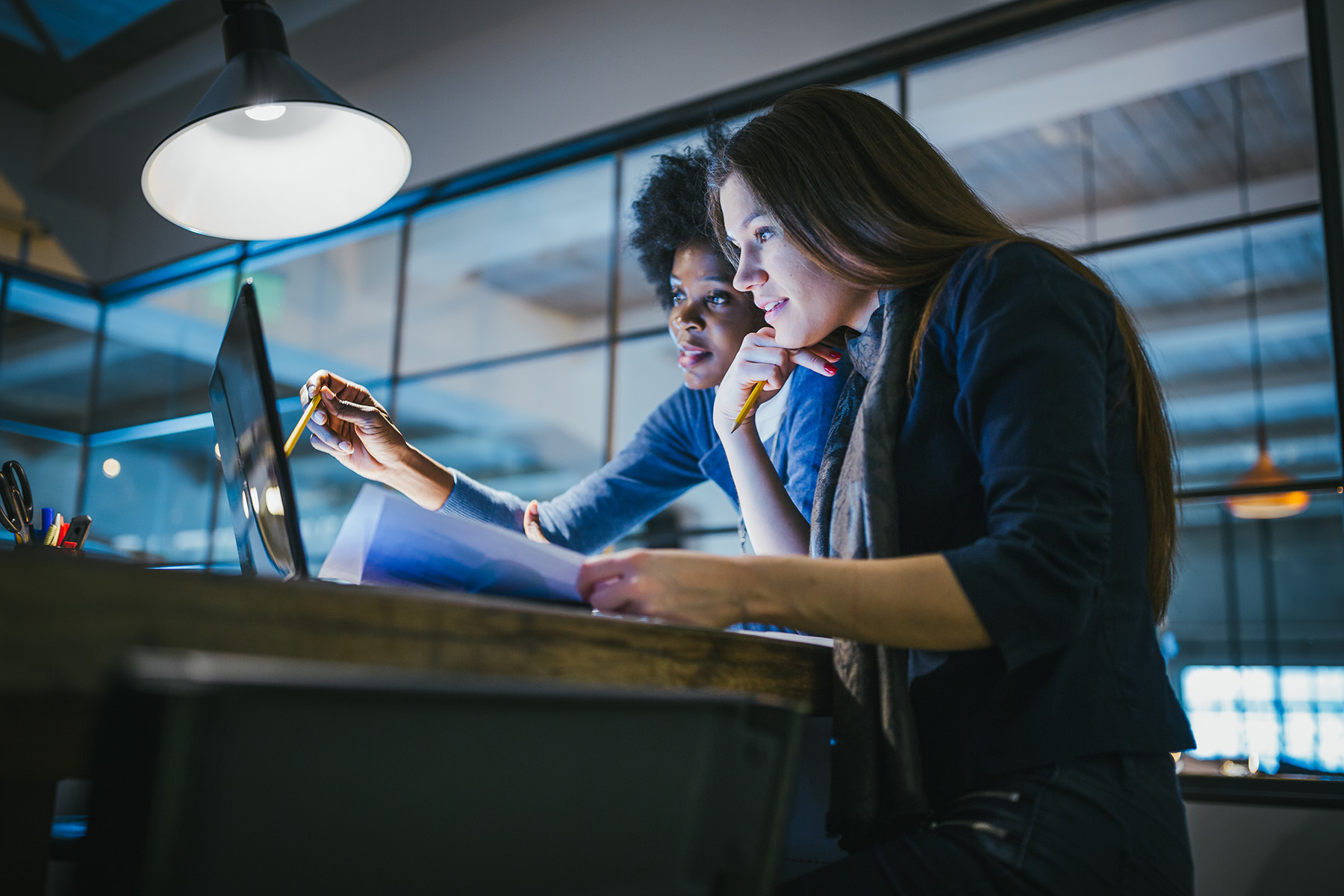
In that regard, AI systems can actually assist in their own investigations because these systems often “give you the resources they based their responses on,” Otani adds. “So, it’s a second step to go to those sources to verify, but it’s a necessary step.”
For Stephen, the verification process is an extension of the quality control effort. For text results, verification should follow a triangulation strategy, examining three primary sources to confirm the AI’s results. Such measures can also help temper the overhyped promises of AI efficiency. For example, an AI system might be touted for reducing the cost of a particular task from $100 if a human engineer does the work to just $5 when the computer takes over.
“But for every AI use, there is an equal quality control innovation that needs to be done,” Stephen says. Adding in the quality control cost might bring the total cost up to $50, which is still 50% less than if the task had been done without using AI, “but that quality control cost needs to be understood and be part of the general conversation,” Stephen explains.
Ethical reviews
AI also presents new ethical issues for civil engineers to consider, from who owns the copyright of AI-generated images, to who controls the data collected or generated by such systems, to potential biases introduced into AI systems by their programmers — consciously or unconsciously.
At Stanford, an ethics and society review board requires researchers seeking funding from the Stanford Institute for Human-Centered Artificial Intelligence “to consider how their proposals might pose negative ethical and societal risks” and to develop methods to lessen them, according to a June 2021 university press release.
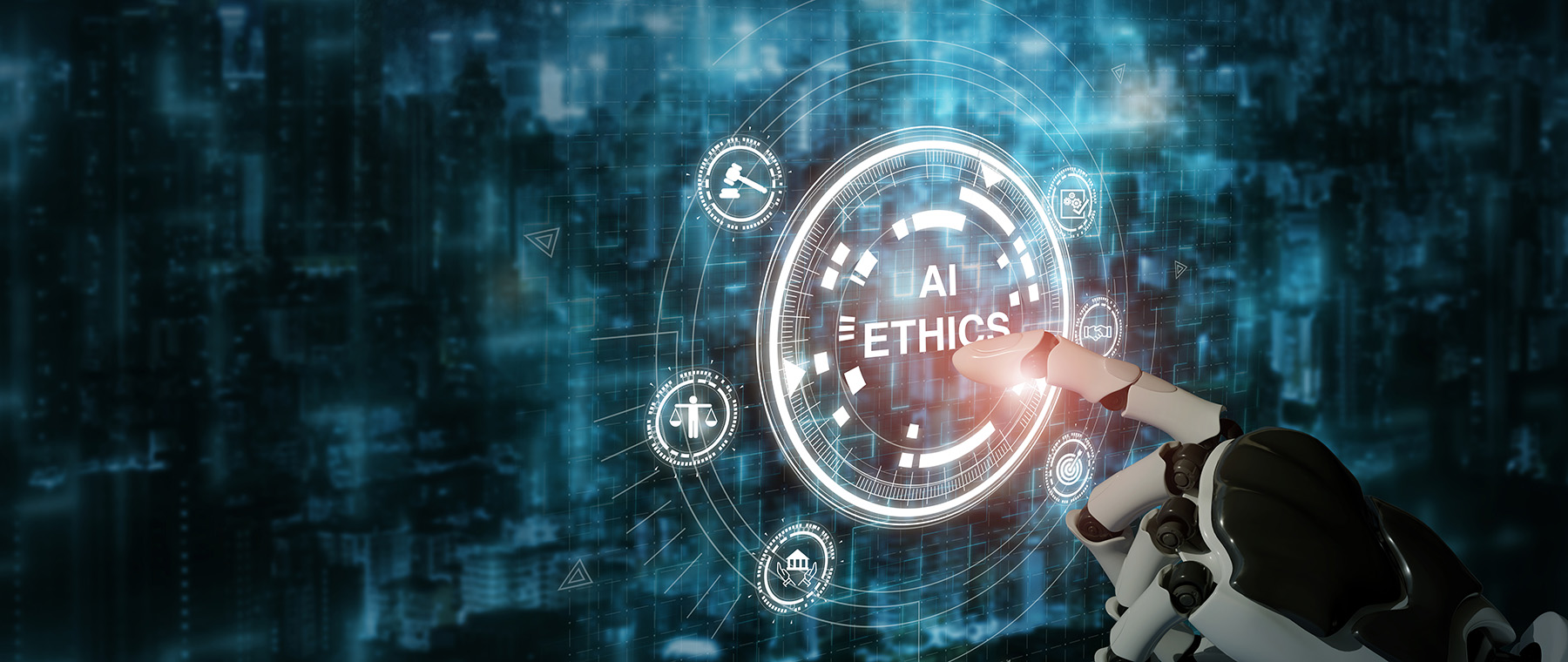
Billington participated in a pilot study with the review board that examined her research team’s work on human-centered building design, harnessing data collection and machine learning to “sense and respond to the well-being of occupants within a structure, including adapting the building systems and digital environments to support and enhance occupant experiences,” the press release noted.
She credits the review board with helping her team consider more deeply the privacy implications of the data they were collecting, which began with an office building setting, expanded to in-home healthcare for the elderly, and now explores the forced labor supply chain issues mentioned earlier. In particular, the review board “asked us how would we use our platform, as researchers at Stanford, to promote ethical, responsible use of AI? That stuck with me,” Billington notes. “So, every time I give a talk now, I talk about the ethical implications and what we’re doing about (them).”
Being the best
The average civil engineer might not need an advanced degree in computer science to succeed in an AI-enabled civil engineering industry, but the more that engineers know about these tools, the more valuable they will be to their employers and their clients.
And it certainly will not hurt anyone’s career to learn basic coding languages or understand programming tools, Odeh notes. Many younger engineers enter the workforce today with such knowledge already in their tool set, he adds, which makes them able to “immediately solve problems in new ways that I think soon will almost be a prerequisite for being an engineer.”
Although there are certain mundane, manual tasks that AI might take over for civil engineers, the real issue is that “AI is not going to replace your job; the person using AI will replace your job!” stresses Stephen. The growing importance of AI skills in civil engineering should eventually lead to some sort of regulation along the lines of the P.E. licensure, Stephen adds.
Likewise, Barua argues that AI governance issues need much more attention because AI-powered products and services “are proliferating at an exponential rate amid the wide arena of regulation-free cyberspace.”
More optimistically, Akinci believes that AI will ultimately “force us to be the best civil engineers we can be.” AI systems will make mistakes because of faulty inputs, undetected or unintended biases, and other factors. Consequently, society’s reliance on civil engineers will increase. “I can’t imagine any system can replace the civil engineer’s intuition, creative thinking, critical thinking, and ethics,” Akinci concludes.
Robert L. Reid is senior editor and features manager of Civil Engineering magazine.
This article first appeared in the November/December 2024 issue of Civil Engineering as “CEs and AI: An Overview.”