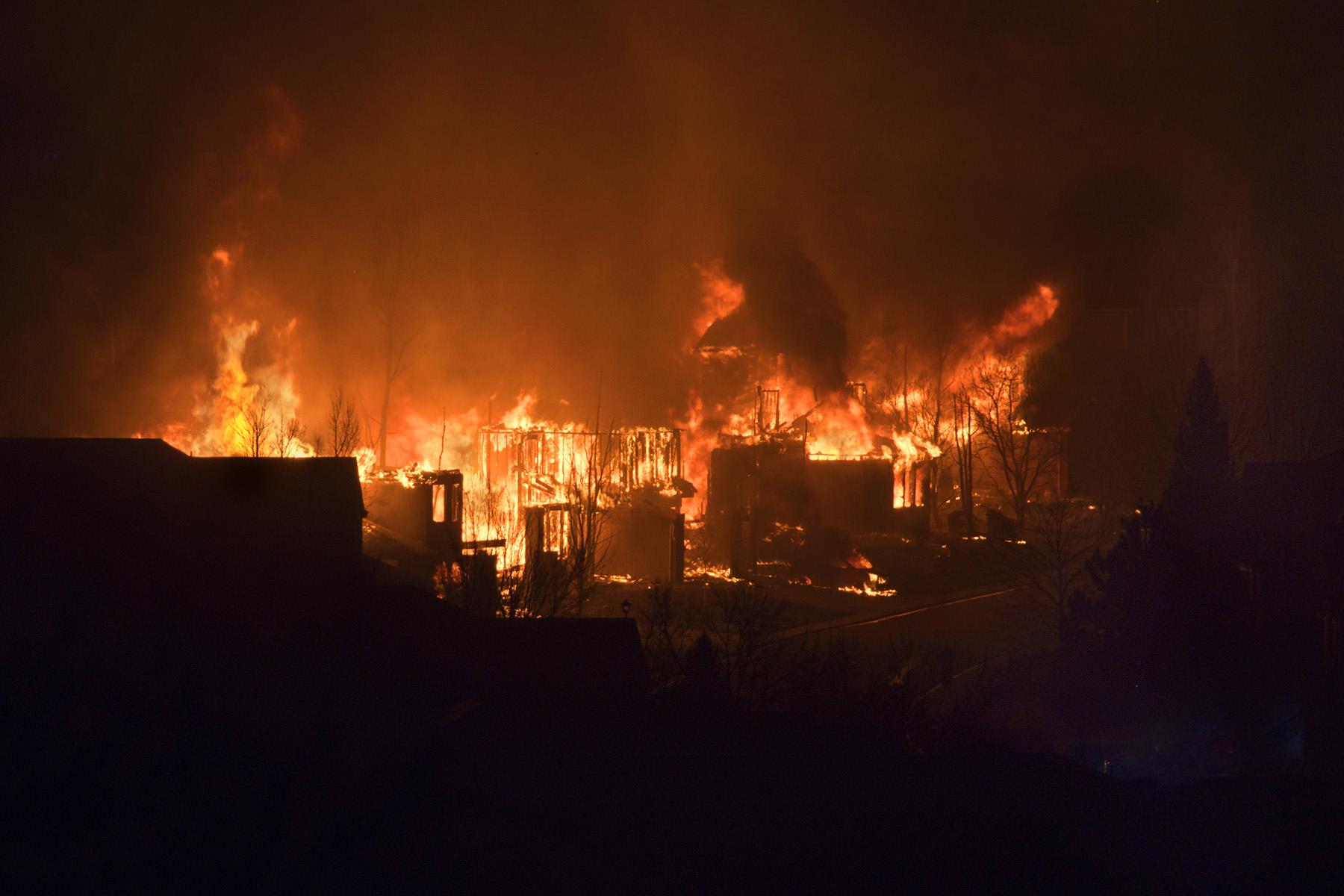
By Hussam Mahmoud, Ph.D., F.SEI, M.ASCE
Researchers have developed a fire propagation model that can determine whether a building will survive a wildfire. It will have profound implications for civil engineers, policymakers, and communities.
Wildfires in the wildland-urban interface, also known as the WUI, have caused unprecedented structural damage worldwide. In the United States, some of the worst wildfires have been within recent history, most notably the 2018 Camp Fire in California, the 2021/2022 Marshall Fire in Colorado, and last year’s wildfires on the island of Maui in Hawaii. Events like these demonstrate the level of destruction wildfires leave in their wake. Mitigating these damages continues to challenge communities, policymakers, insurance companies, and engineers.
Controlling structural damage during earthquakes, for example, is possible because there is sufficient knowledge of what factors influence the intensity of earthquakes and the structural response. For wildfires, it has not been so simple. Wildfire propagation models have been in use for years, but they are limited in that they have not been able to estimate structural damage with much success. But researchers at Colorado State University have developed a computationally efficient model, dubbed the Asynchronous Graph Nexus Infrastructure for Network Assessment of Wildland-Urban Interface Risk, or AGNI-NAR, that can predict structural damage and accurately trace how a fire will run through a community.
Immediate interconnectivity
WUI fires are distinct from other threats — earthquakes, hurricanes, and floods — as each burn of a structure or vegetation increases the hazard’s intensity. Whether a building catches fire depends on its properties and on whether nearby vegetation and structures are already burning. Ignition of these nearby objects will further depend on the properties and conditions of any directly adjacent buildings or vegetation. The complex interconnectivity between vegetation and a structure allows the fire to initiate and spread using different mechanisms — conduction, convection, radiation, or ember spotting.
The impact of this interconnectivity on wildfire spread and damage is not embraced by policymakers when assessing wildfire behavior. Early studies on wildfire behavior in communities emphasized short-range interactions between a structure and its immediate environment, resulting in the adoption of the home ignition zone concept.
The HIZ is a small area (approximately 100 ft by 100 ft) that comprises the structure itself as well as the surrounding area, including ignitable building materials (decks, fences, etc.), flammable debris, and vegetation. Existing fire mitigation policies are based on the HIZ concept, which looks at the vulnerability of a home as influenced by its immediate surroundings but not by homes and vegetation that are farther away.
This method of assessing a home’s vulnerability is based on the idea that by reducing the home’s susceptibility to catching fire, the chances of said structure surviving an ember storm or spot fire increase. (Embers are small pieces of burning material generated from trees and/or homes. These embers can be carried by the wind. A spot fire is a fire that ignites from embers.) This is a valid contention for communities in which vegetation and homes are not close to each other, allowing embers to be ignition’s main driving mechanism.
However, for communities with limited vegetation (Marshall Fire) or where homes and/or vegetation are narrowly spaced (Camp and Marshall fires), other mechanisms such as structure-to-structure or vegetation-to-structure ignition could be the main drivers for fire spread.
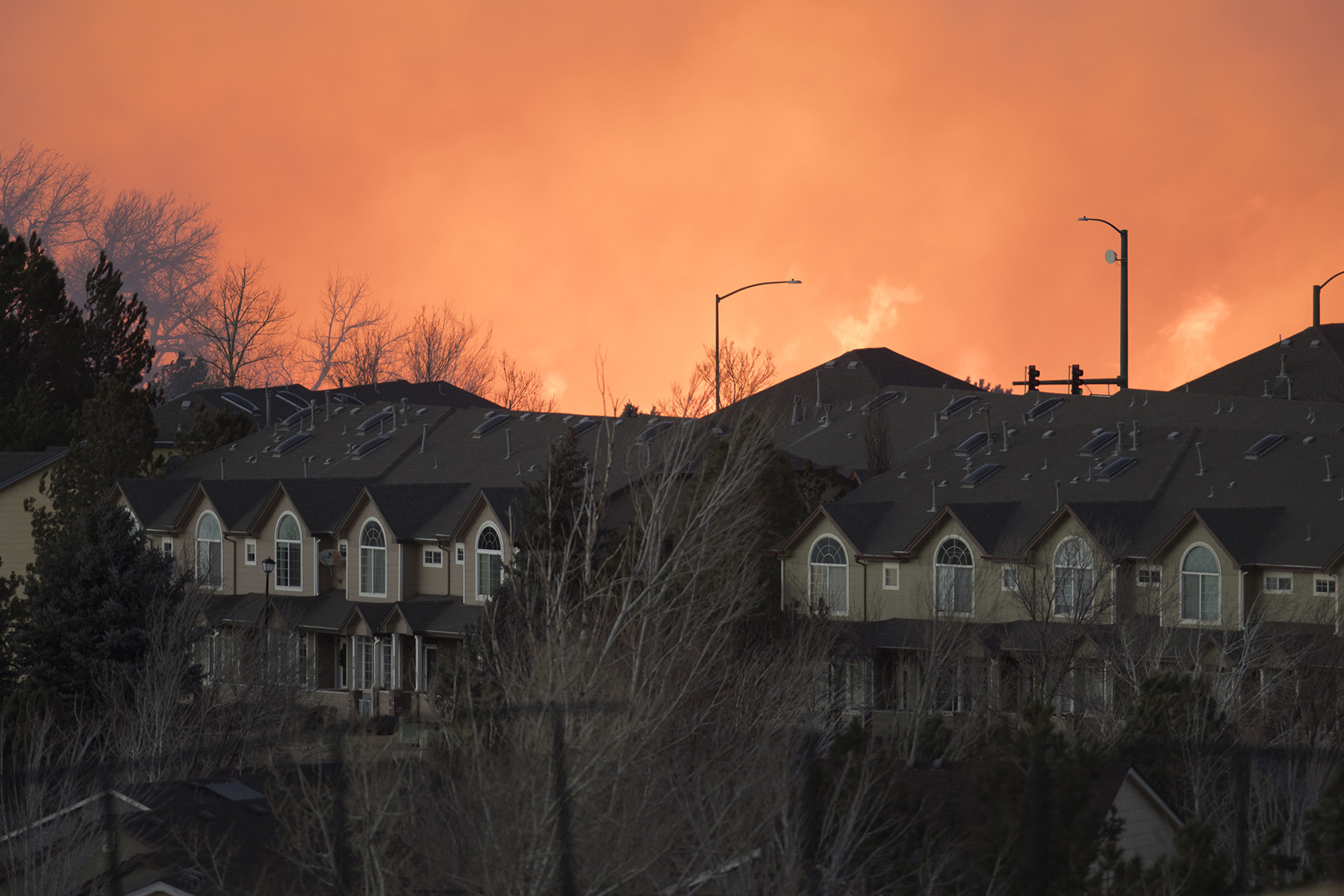
As stated above, wildfire propagation models are in use by fire management agencies and researchers, encompassing various types, from empirical to entirely analytical. These models, although widely accepted, focus mainly on wildland fires. Robust computational fluid dynamic fire-spread models exist and can be used to capture the fire interaction between buildings and vegetation. However, their complexity and computational demands limit their application on a community scale. As computational infrastructure advances, the profession may eventually rely heavily on computational fluid dynamic models, but the escalating risk of WUI fires necessitates immediate action.
Therefore, it is crucial to explore efficient modeling approaches for quantifying how wildfires spread in communities.
A brief history
The need for accurate and computationally efficient WUI fire propagation models prompted the Socio-Physical and Hazard-Integrated Environment, or SoPHIE, research group (which I lead) at Colorado State University to find an alternate approach to simulate wildfire propagation in communities. The group sought to develop a model, now known as AGNI-NAR, to capture the fire interaction between structures and vegetation as a network. Capturing this interaction would allow the team to determine how removing vegetation and hardening a structure (lowering its chance of ignition) might impact damage potential for an entire community. The model would also help determine how to allocate and position firefighting resources or even the best evacuation routes.
The effort to develop this wildfire simulation approach began in 2015 with a five-year grant from the National Institute of Standards and Technology to develop the science for risk-based community resilience planning. Part of this grant was allocated to study wildfire propagation as well as for the partial support of then-CSU student Akshat Chulahwat. We were the only two researchers working on the WUI problem within the NIST-funded Center for Risk-Based Community Resilience Planning.
In 2020 the center received funding for another five years. However, the group’s focus turned to integrating social science with other hazards, like earthquakes and floods, that were further along regarding hazard assessment and damage predictions. As a result, we did not have the financial support to continue our work on predicting damage due to WUI fires. Chulahwat, now a postdoctoral scholar in the SoPHIE research group, decided to return to India, but we continued the work on the AGNI-NAR model with no funding. We met online every week to discuss our progress. We knew we were on to something, so we were persistent.
In 2022, Chulahwat and I, along with others, published the paper “Integrated graph measures reveal survival likelihood for buildings in wildfire events” in Scientific Reports, which showed for the first time how the AGNI-NAR model results compared to damage from actual wildfires. The results sparked the interest of many stakeholders and philanthropists, including the Gordon and Betty Moore Foundation, which awarded us a $2.7 million grant. With this grant, my team and I are now working on many developments, including enhancing our initial wildfire spread model, automating the extraction of building and vegetation features using deep learning (a type of machine learning inspired by the structure of the human brain), determining local wind fields in communities, capturing time-dependent fire propagation, and translating the research into practice.
Although the newly funded project focuses on damage assessment, many opportunities exist to integrate our work with other critical aspects influencing wildfire vulnerability and risk. For example, there is clear evidence that the wildfire season is getting longer, and its intensity is increasing. Both factors will impact vulnerability and risk.
Double analyses
The research team’s focus is also on enhancing wildfire vulnerability analysis. AGNI-NAR predicts the fire boundary and the structural vulnerability across a community. The boundary analysis provides predictions as to which region will encompass a fire by determining the likelihood of a structure or vegetation being in the path of the fire. The vulnerability analysis provides an estimate of the likelihood of a structure surviving or being damaged in a wildfire event. The model is also applicable to other infrastructure as long as the structural location and construction material are known.
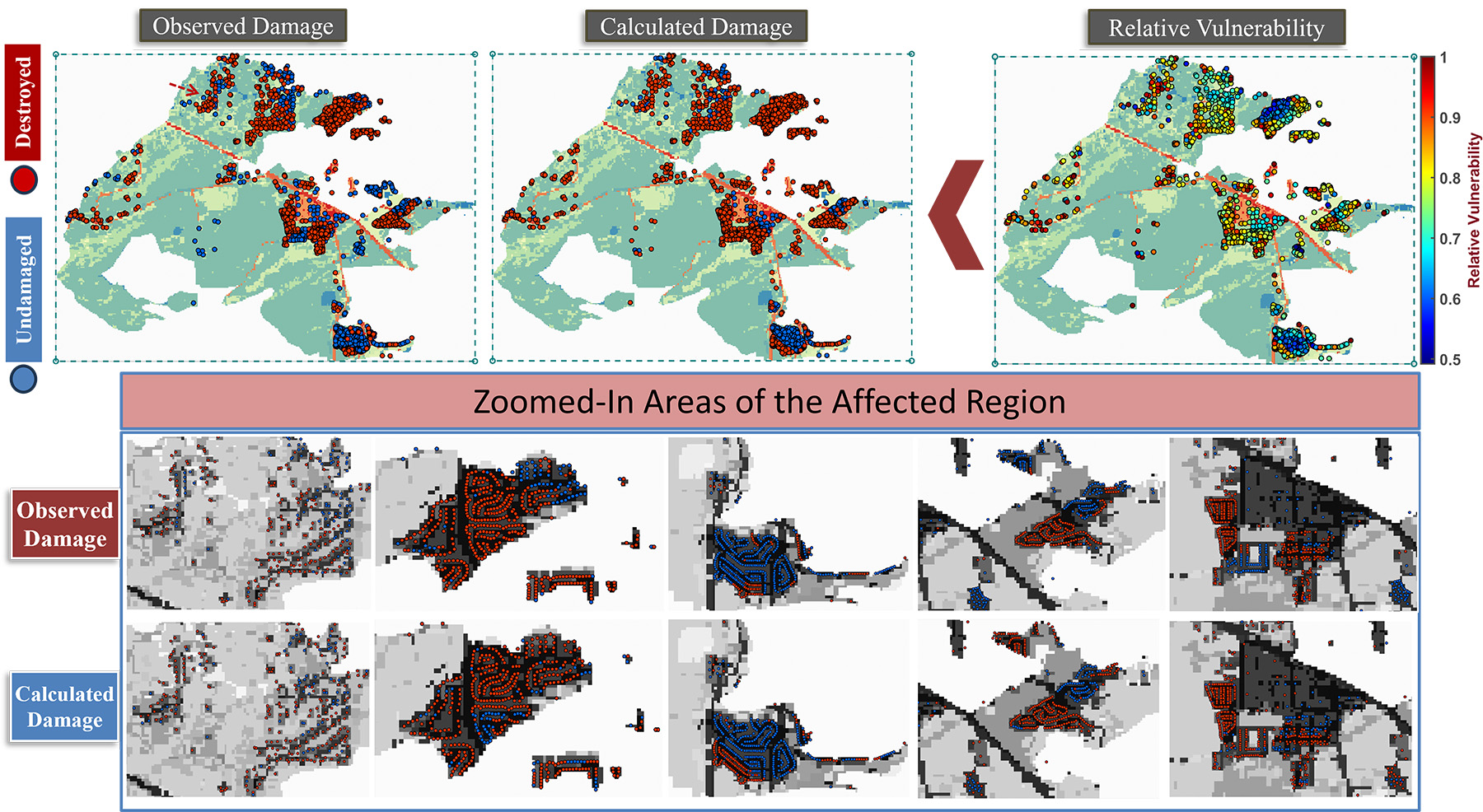
Many factors influence the potential for a fire to reach and ignite a structure during a wildfire event. These factors vary from one structure to another and from one community to another. Because all buildings and vegetation are connected as a network, and every community has a different building and vegetation layout, factors necessary for determining damage across one entire community will not be the same as controlling factors for another community. Recognizing this fact is key to lowering wildfire damage in communities. The reality is that no one-size-fits-all solution exists, and tailored solutions have to be developed for each community.
For buildings, many factors impact the potential for wildfire damage, including roof material, window type (single or double pane), and siding material. But these are not the only important factors. The location of a building relative to other buildings and vegetation in a community, the location and orientation relative to the dominant wind direction, the presence of vegetation near the building (i.e., fuel in the defensible space), and the proximity to the wildland are also critical factors. A building or vegetation in one location could cause ignition of a building or vegetation in another location within the community.
Propagation and transmission
Recognizing the need to establish a computationally efficient framework that can capture the fire interaction between buildings and vegetation in a network setting, we shifted our attention to graph theory. Graph theory is a mathematical discipline that analyzes the relationships among interconnected elements. It has emerged as a versatile tool to understand network behavior across scientific domains, and it has found various applications in many disciplines, from the ripple effects of economic failures to disease transmission in a social or ecological setting.
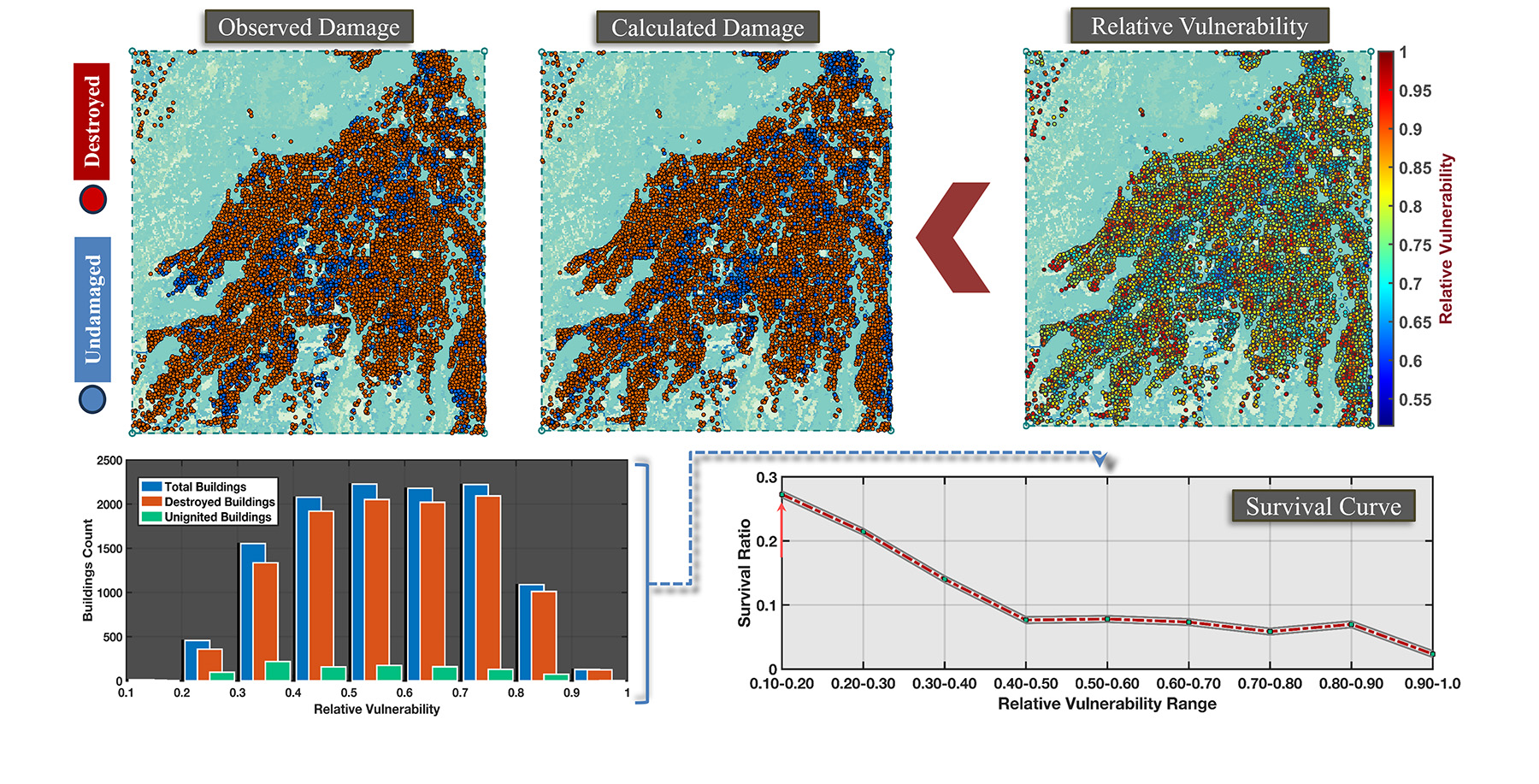
Our paper “Unraveling the Complexity of Wildland Urban Interface Fires,” published in 2018 in Scientific Reports, references how wildfire propagation in communities is similar to disease transmission. Still, not until COVID-19 hit in 2020 did we realize that similarities between wildfire propagation and disease transmission could be used to understand even more about the AGNI-NAR model.
Infectious diseases spread from person to person based on several factors, such as immune system strength and the likelihood of a person coming in contact with infected individuals. The contagious nature of pandemics compelled communities to implement measures to combat the spread of COVID-19, including contact tracing, mask mandates, vaccinations, social distancing, and border closures.
Wildfire mitigation measures and how they impact fire spread in entire communities can be viewed analogously. For example, mapping the areas that surround structures is similar to contact tracing; hardening buildings by using fireproof roof material is akin to mask and immunization mandates; clearing vegetation to create defensible space is reminiscent of social distancing; and establishing buffer zones at the WUI is similar to border closures.
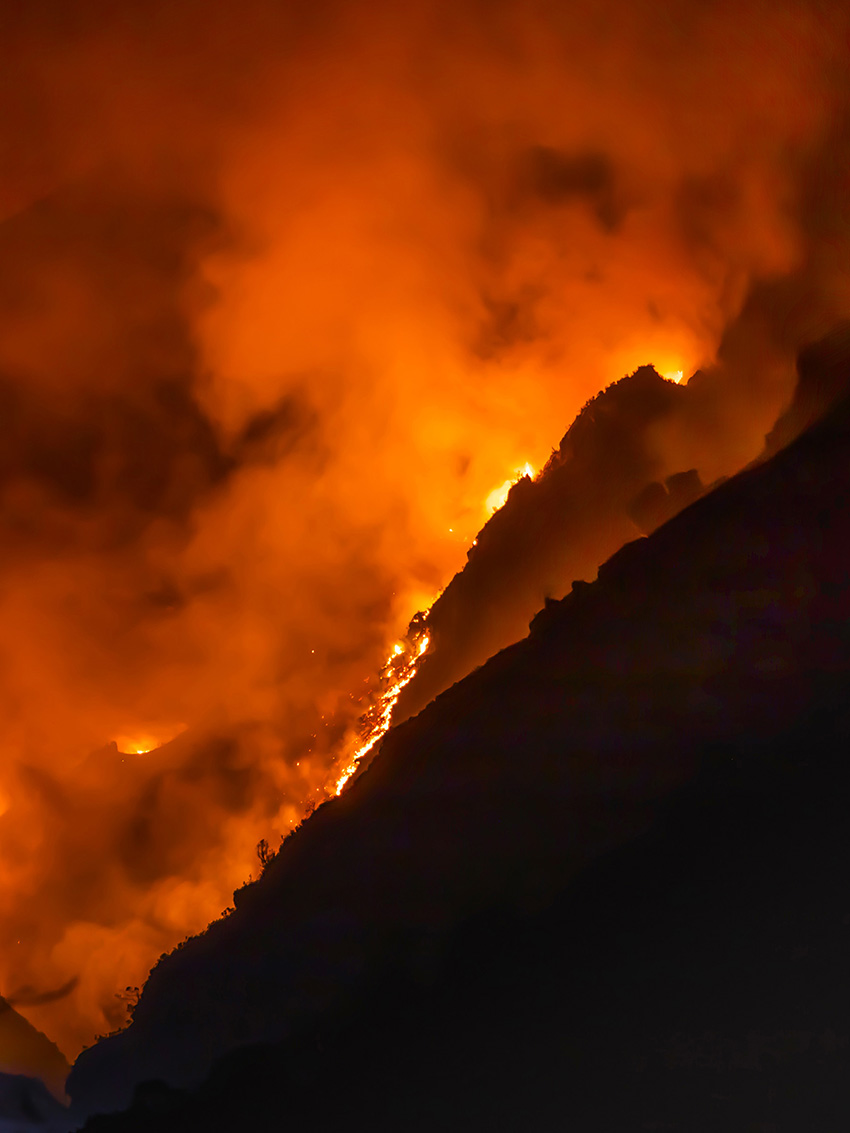
The AGNI-NAR model relies on existing data on buildings, vegetation, and wind fields, and it can predict whether a building will survive or burn in a wildfire event with those data inputs. For buildings, the model uses existing layers of building footprints mapped from high-quality geospatial satellite images such as the data provided by Microsoft or the U.S. Army Corps of Engineers.
For some community buildings, individual attribute data also exist, including roof type, siding material, and deck and fence material. For example, the California Department of Forestry and Fire Protection records building features through the Damage Inspection Specialist dataset for communities that have experienced actual fires.
For vegetation data, the model uses surface and canopy fuels from LANDFIRE, a program that provides “geospatial data and databases that describe vegetation, wildland fuel, and fire regimes across the United States and insular areas,” according to its website.
Obtaining data for fuel in the defensible space (within 100 ft of a building) is challenging. However, some cities and counties are outsourcing such data collection. Wind data were collected from the National Oceanic and Atmospheric Administration database.
To date, the AGNI-NAR model has been tested on four historical fires: the Camp, Glass (2020), Marshall, and Maui fires. The analyses of the four fires resulted in an accuracy of approximately 85%, 64%, 72%, and 74%, respectively.
The results are sensitive to the wind field; therefore, reliance on accurate wind simulations at acceptable resolutions will improve the outcome. Due to the lack of data, factors not considered in the analyses included firefighting or intervention efforts and the presence of wood fences or cars near homes.
For the future
The ability of the AGNI-NAR model to capture the likelihood of building damage in a wildfire event opens up a new paradigm for tackling the wildfire problem. We can now reverse-engineer the problem and determine which buildings should be hardened, as well as which vegetative fuel — in the wildland and in communities — should be reduced, and to what extent, in order to lower the potential for damage across a community.
The model can also establish optimal evacuation routes and firefighting strategies and aid in the design of new communities. The model-building process is being automated to allow engineers and policymakers to use it more efficiently.
Requiring every household in wildfire-prone areas to adhere to existing policies is not practical, and leaving the decision of whether to implement mitigations to policymakers undermines the purpose of having policies in the first place. Current wildfire policies are not enforced, nor do they consider how applied mitigations at one structure or tree might impact damage to the whole of the building portfolio. Undoubtedly, the scope of wildfire mitigation policies needs to expand to encompass the behavior of a structure/vegetation in a network setting.
Accurate and computationally efficient wildfire models are required to understand how fires spread in communities. With urbanization and population growth rising, there must be effective mitigations to coexist with wildfires. The road ahead is long and challenging, but together, we can solve the complex wildfire problem.
Hussam Mahmoud, Ph.D., F.SEI, M.ASCE, is the George T. Abell Professor in infrastructure and the director, structural laboratory in the department of civil and environmental engineering at Colorado State University. He also leads the Socio-Physical and Hazard-Integrated Environment.
This article first appeared in the July/August 2024 issue of Civil Engineering as “Taming Fire.”
References
Chulahwat, A., Mahmoud, H., Monedero, S., Diez Vizcaíno, F. J., Ramirez, J., Buckley, D., & Cardil Forradellas, A. (2022). Integrated graph measures reveal survival likelihood for buildings in wildfire events. Scientific Reports, 12 (Article 15954). https://doi.org/10.1038/s41598-022-19875-1
Mahmoud, H. & Chulahwat, A. (2018). Unraveling the complexity of wildland urban interface fires. Scientific Reports, 8 (Article 9315). https://doi.org/10.1038/s41598-018-27215-5
Further reading
“Leveraging epidemic network models towards wildfire resilience” by Hussam Mahmoud, Ph.D., F.SEI, M.ASCE www.nature.com/articles/s43588-024-00619-2