Prepared by Mehzabeen Mannan, Monzure-Khoda Kazi, Upadhyayula Venkata Krishna Kumar, and Venkataramana Gadhamshetty and submitted to the Fall 2024 Currents.
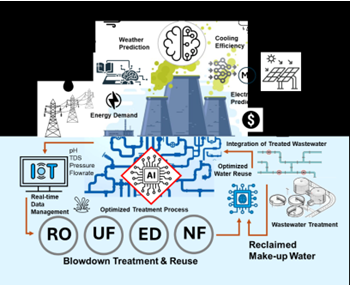
Artificial Intelligence (AI) has transformative potential for addressing water challenges related to accessibility, quality, and governance, especially under pressures from natural hazards, climate change, population growth, and resource scarcity. AI is particularly effective within the energy-water nexus, where issues are acute in drought-prone regions like the western United States. This region relies heavily on thermoelectric power plants, which require substantial water withdrawals for cooling. With climate projections indicating increased drought risks, dependence on these plants will grow, further depleting freshwater resources and raising thermal pollution levels. Traditional water-intensive cooling systems in these plants thus threaten sustainable water management in regions vulnerable to drought.
AI, combined with the Internet of Things (IoT), can transform water management by predicting system failures, optimizing treatment, and improving reuse strategies (figure above). Using real-time data, AI-driven systems boost cooling technology efficiency, essential in water-stressed regions, aligning with EWRI’s mission to promote water conservation and resilience. AI also enhances the design of energy-efficient cooling systems in industries facing strict regulations and high costs. The cooling tower market, worth $622.5 million in 2023, is projected to reach $933.09 million by 2032, with many systems relying on water-intensive evaporative cooling. These systems increase dissolved salts and contaminants, risking scaling and equipment damage. AI-based systems offer an opportunity to minimize water use while maintaining cool efficiency through predictive modeling of system performance and real-time water chemistry management. By optimizing water recirculation, blowdown, and make-up, AI reduces energy consumption and environmental impact. Additionally, AI integrates renewable energy by adjusting operations to real-time availability, improving energy efficiency and supporting sustainability goals.
Wastewater reuse in power plants and the role of AI
Integrating AI with advanced water reuse technologies offers a path to optimizing both water and energy resources, driving sustainability in cooling systems, and supporting broader goals of water conservation and resilience. As water scarcity continues to drive industrial innovation, treated wastewater, such as municipal reclaimed water, has emerged as a viable alternative to traditional freshwater sources for cooling systems. For instance, a study at an oil and gas facility in Saudi Arabia demonstrated the use of treated wastewater effluent from an activated sludge system in a 4.2 MW cooling tower, which reduced water consumption by 27%. The use of reverse osmosis (RO) membranes for treating reclaimed wastewater further enhanced efficiency, though membrane fouling remained a challenge. Here, AI plays a critical role in both monitoring and predicting membrane performance by analyzing water quality parameters such as TDS (Total Dissolved Solids), flow rate, and pressure differentials. By applying machine learning models, AI systems can detect early signs of fouling, enabling timely maintenance and operational adjustments to maximize membrane lifespan. AI can monitor and control treatment conditions, optimize the use of RO membranes, and address fouling issues by providing guidance on needed surface modifications of the ultrafiltration (UF) membranes with monomers like BET SO3- and N(CH3)3+. Additionally, integrating microbial fuel cells (MFC) with modified UF membranes for wastewater treatment and reuse eliminates the need for external aeration, achieving a 90% reduction in chemical oxygen demand (COD) while recovering energy in the form of bioelectricity, further reducing the overall operational cost. This produces high-quality cooling water that met Electrical Power Research Institute (EPRI) standards. Other technologies for cooling water blowdown (CWBD) treatment include membrane distillation (MD), ballasted sand flocculation (BSF), vibratory shear enhanced membrane process (VSEP), electrocoagulation (EC), nanofiltration (NF), electrodialysis (ED) are also being explored for sustainable water management in power plants. AI has the potential to integrate these processes, providing a real-time decision-making framework that optimizes treatment selection based on water quality, energy usage, and economic feasibility.
Recent research into advanced Cooling Tower Blowdown (CTBD) highlights water conservation benefits. Managing cycles of concentration (CoC) is key: excessive CoC leads to scaling and corrosion, while low CoC wastes water. Lime softening can recycle CTBD by blending up to 25% with make-up water without extra CaO, ensuring compliance. AI can optimize this by dynamically adjusting CoC in real-time, enhancing water reuse and preventing scaling. Constructed wetlands (CWs) and zero liquid discharge (ZLD) systems are also effective for CTBD treatment, with CWs reducing chemical costs and ZLD systems providing high-quality effluent while minimizing water withdrawals. Techno-economic analyses suggest blowdown reuse is most feasible for systems operating at CoC levels above 3, offering 13% water savings and lowering costs (14). AI can enhance this process with real-time insights, enabling optimal CoC management for greater water recovery and a reduced environmental footprint.
AI has been applied in numerous studies to optimize cooling tower performance, targeting parameters like air velocity, water flow rate, and nanoparticle concentration, evaporated water mass estimation, thermal performance under crosswind conditions, legionnaires’ disease outbreak investigation, and energy discharge level prediction, tracking and tracing water consumption, long-term water conservation, and activated sludge modeling. In power plants, these AI applications are essential for operational efficiency, regulatory compliance, and minimizing environmental impact.
Conclusion
Optimizing water management with AI requires integrating predictive modeling, water treatment, and energy efficiency, particularly in power plant cooling towers. AI systems can analyze real-time sensor data on water quality, flow rates, and microbial activity to optimize CTBD recycling or discharge for cooling efficiency and compliance. By assessing salinity, contaminants, and microbial loads, AI can enhance wastewater integration, balancing operational efficiency with energy conservation. Additionally, AI can reduce energy use by monitoring electricity prices and prioritizing renewables during peak times. Forecasting cooling demand based on weather, AI aligns water and energy strategies in real time, minimizing environmental impact. However, challenges persist in integrating AI with existing infrastructure, managing diverse data sources, and ensuring cybersecurity. AI models must handle variable water quality, fluctuating energy demands, and stringent regulations. For effective implementation, industries should invest in AI-compatible infrastructure, train personnel, and collaborate with research institutions. Experts in water and environmental engineering can support this transition by developing standards, assessing impacts, and providing guidance. Leveraging AI-driven insights and expert guidance allows power industries to optimize water and energy use, reduce environmental impact, and achieve sustainability goals, ultimately enhancing operational efficiency, reliability, and resilience of water resources.
For information related to references or authors' organizations, please contact EWRI.